Another month, another exciting AI round-up!
In this month’s edition, we’ll cover a few topics in AI and machine learning, from healthcare to aerospace.
Read on to learn how:
- AI could help narrow the heart attack gender gap
- AI shines a light on the Moon’s permanently shadowed regions
- This ‘unprecedented’ AI chip could revolutionize AI
- Gallium oxide’s crystalline complexity was tamed by machine learning
- Machine learning could revolutionize mineral exploration
AI could help narrow the heart attack gender gap
The BHF (British Heart Foundation) found in funded research that women in the UK who have a heart attack don’t receive the same quality of care as men. Women are 50% more likely to have a wrong initial diagnosis, as current guidelines to diagnose heart attacks use the same threshold for all patients.
BHF-funded research from the University of Edinburgh merged data from 10,038 people, 48% of them women, who were admitted to hospital with a suspected heart attack and developed an AI-based tool to help medical professionals diagnose heart attacks more accurately.
The AI-based tool, CoDE-ACS, combines patient information that’s routinely collected at the hospital with the protein troponin, which is the gold-standard for heart attack diagnoses. It then produces a score between 0 and 100.
The researchers found that the tool could rule out a heart attack with an accuracy of 99.5%, while also identifying the patients who benefitted from further tests, in whom the final diagnosis of a heart attack had an accuracy of 83.7%. This is an improvement from the current 49.4% accuracy of present tests.
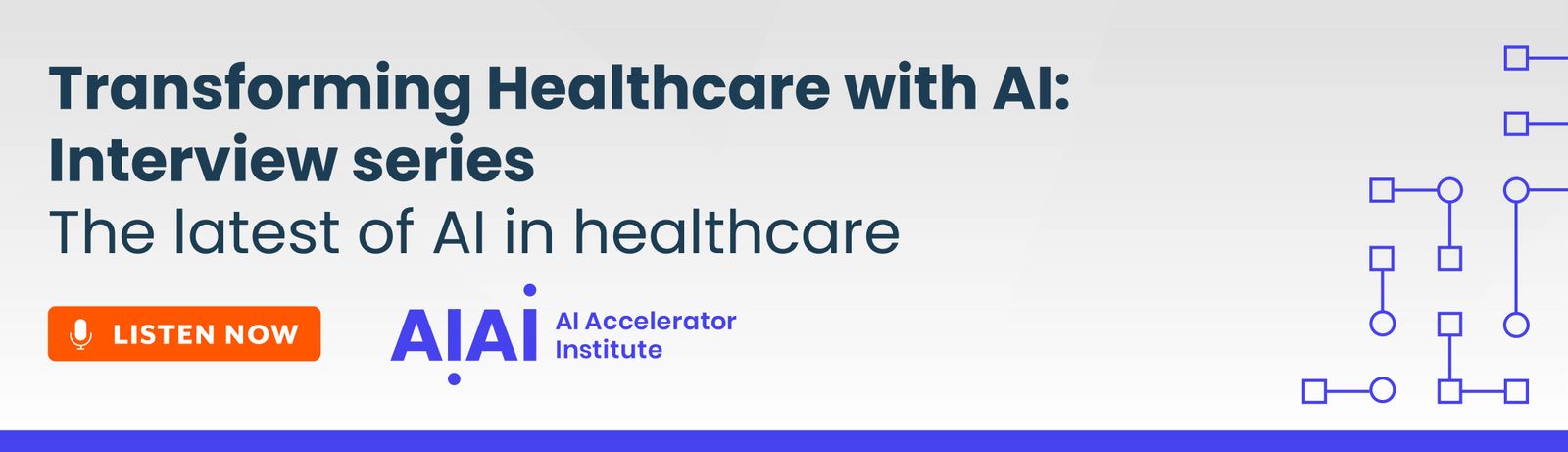
AI shines a light on the Moon’s permanently shadowed regions
NASA’s future lunar missions have the Moon’s darker regions as targets, as they have the potential of harboring water ice – essential to break down into oxygen and hydrogen for both fuel and breathing air. As these dark regions have temperatures as low as -274℉-400℉, they trap ice by preventing it from sublimating into a gas.
Glaciologist Dr. Valentin Bickel of ETH Zürich and his colleagues used images from NASA’s Lunar Reconnaissance Orbiter in their study. The spacecraft’s camera captures photons that are bounced into the shadowed areas of the Moon, and AI helps the team to make efficient use of the data, which revealed that there’s no visible water ice in these locations - even though other instruments have detected its presence.
This implies that any existing ice has to be mixed with lunar soil or be present under the surface.
‘Unprecedented’ AI chip could revolutionize artificial intelligence
A new AI system could help complex artificial intelligence live on chips – all without having to send any information to the cloud. Most AI applications don’t run locally on devices because of limited battery life and processing power. Instead, data is sent over the internet to other computers to be analyzed and sent back.
This new breakthrough in AI tech is a step towards having artificial intelligence embedded in edge devices for faster and more efficient performance. It uses a “resistive random access memory”, which lets computation be performed directly on the memory.
The system showed a 99% accuracy when it analyzed handwritten digits and an 84.7% accuracy on a Google voice recognition task.
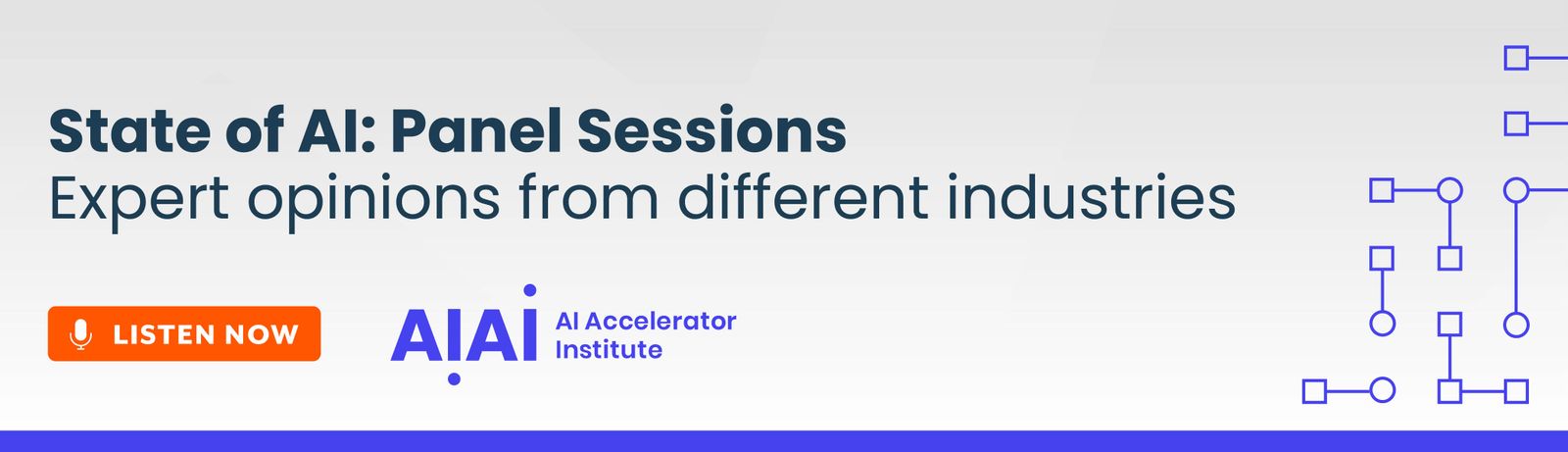
Gallium oxide: Crystal complexity tamed by machine learning
Researchers from the University of Bristol, the University of Liverpool, University College London, Diamond Light Source, and countries like Italy, Germany, Japan, and the USA, have created a new understanding of gallium oxide, made possible by combining experimental results with a machine learning theoretical approach.
Gallium oxide has promising applications in both solar-blind photodetectors and power electronics, but it provides a challenge across synthesis, characterization, and theory because of its inherent disorder and resulting complex structure (electronic structure relationship).
Gallium oxide has five different phases, or crystal structures, which are alpha, beta, gamma, delta, and epsilon. It’s also got four inequivalent gallium lattice sites, which are partially occupied in an inherently disordered structure. This means it has a deceptively simple cubic symmetry but it’s immensely complex. With an enormous number of possible crystal structures, it makes conventional theoretical approaches impossible.
First principle calculations are combined with machine learning to filter almost one million possible structures in 160-atom cells. Dr. Laura Ratcliff from the University of Bristol’s Centre for Computational Chemistry, the lead author of this study, said: “(...) The predicted low energy configurations provide a good description of the experimental data, whilst clear deviations are found for the higher energy configurations, confirming that these are not a realistic description of the disorder in gamma-gallium oxide.”
Machine learning could revolutionize mineral exploration
There’s been a decrease in the discovery rate of porphyry deposits, as the mining industry has already identified – and mined – the world’s largest sites. Zou et al, in a recently published study, have presented two novel machine learning techniques to allow for the identification of deeply buried porphyry copper deposits by characterizing magma fertility.
As fertile magma can form porphyry deposits, the researchers aim at improving the traditional geochemical indicators that have high false-positive rates. The algorithms, random forest and deep neural network, were formulated by using a global dataset of zircon chemistry – used to evaluate porphyry copper deposits in magma.
The models focused on 15 trace elements, being validated by independent datasets from two well-characterized porphyry copper deposits in Canada, China, Tibet, and south-central British Columbia.
The models provide a classification accuracy of 90% or higher, with the random forest model having a false-positive rate of 10% and the deep neural network having a false-positive rate of 15%. This is a big improvement from traditional methods, which have false-positive rates between 23%-66%.