Have you wondered: what problems can AI solve?
We’ll look at issues that artificial intelligence can help you solve in your company, specifically the problems highlighted in our AI Software of Choice report.
Image and video recognition was highlighted as the most important problem that AI can solve in a company, having 41.8% of votes from respondents. Customer support at 29.8% and fraud at 10.4% were indicated as the second and third problems solved, respectively.
Before you dive in, can you spare a few minutes to share your expertise in generative AI and help shape the industry?
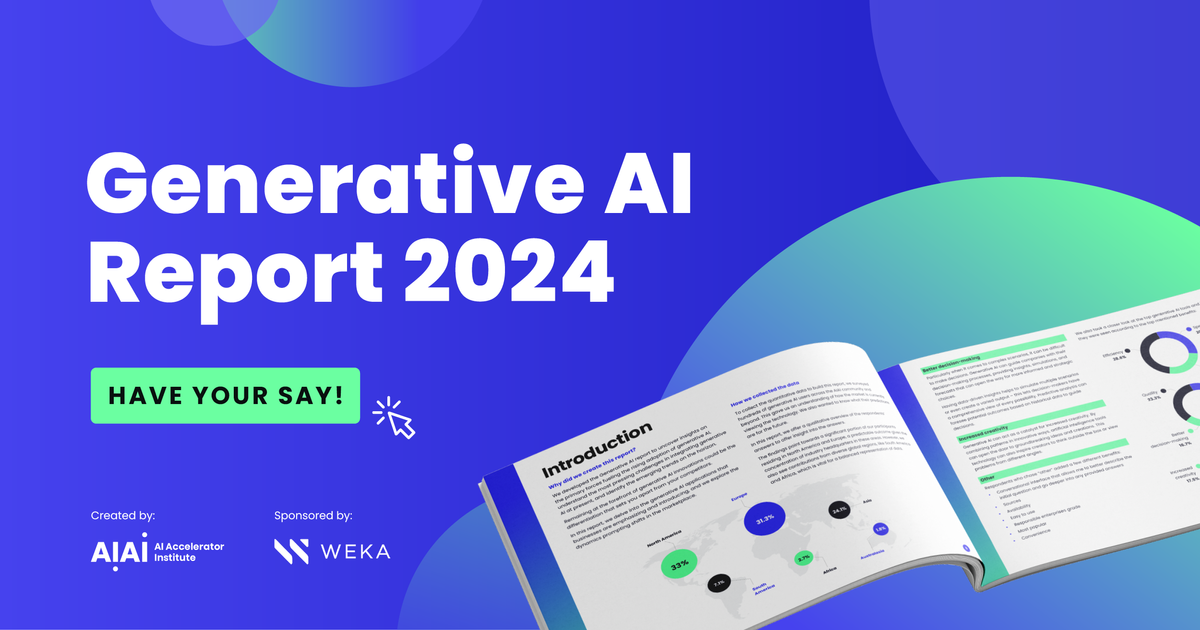
Now, let’s take a closer look at problems AI can solve in companies:
- Customer support
- Data analysis
- Demand forecasting
- Fraud
- Image and video recognition
- Predicting customer behavior
- Productivity
1. Customer support
Businesses that not only know their customers but also cater to their needs tend to come out on top. AI is advancing at an exponential rate, with companies having many ways to use it to learn more about their customer base. AI in customer support offers faster solutions and 24/7 availability, unlike traditional business models.
AI can enhance customer support through solutions such as:
Chatbots
Automating Frequently Asked Questions (FAQs) through chatbots, or conversational AI, can help improve the user experience alongside lowering business costs. Customers benefit from these chatbots having access to information in real time, storing large amounts of data, and predicting customer behavior.
Text and NLP analysis
With Natural Language Processing (NLP), businesses can determine whether a customer’s writing offers a neutral, positive, or negative perception of a specific product or service. It also allows companies to highlight product suggestions and complaints to proactively address issues.
Biometrics
Relating to body measurements and calculations for authentication, access control, and identification purposes, biometrics analyzes specific parts of the human body (e.g. fingerprints, iris) or other characteristics (e.g. voice) to provide higher digital security.
Voice and face recognition
Through the use of biometrics such as voice and face recognition, companies can recognize customers and greet them in a personal way, alongside authenticating them and minimizing the risk of fraud.
Predictive personalization
Offering personalized recommendations and services, predictive personalization uses viewing history, demographic information, personal preferences, and other data to predict what customers will want next.
Intent prediction
Views, clicks, and purchases are transformed into predictions for valuable personalization before customers request them. Figuring out the next steps in specific customers’ buyer journeys can help increase sales. By combining AI with customer data, predictive solutions can also deliver relevant information in customer support.
2. Data analysis
Data analysis is the visualization, manipulation, and transformation of data to infer meaningful insights. A focus on analytics allows businesses to delve deep into their data to improve bottom lines, efficiency, and competitive advantage. Incorporating AI in data infrastructure is only the first step, as it becomes essential for decision-makers, helping to predict stock prices and customer behavior.
AI can enhance data analysis through solutions such as:
New insights
Through machine learning algorithms, data can automatically be analyzed and insights generated. Processes that used to take days or even months can be done in a blink of an eye. AI can spot patterns in large datasets and even recommend actions according to the opportunities it spots.
Data unification
It can be beneficial to have all customer data in a single and unified view, something that AI can help with by pulling data together from different sources to streamline business operations.
Marketing outcomes and business prediction
By analyzing data from numerous sources, AI-powered systems can offer valuable predictions about what works and doesn’t work in your business.
Report generation and dissemination
Insights can be generated rapidly, practically in real-time, which can then be used to automate report writing to allow for human employee relocation. These reports can then be just as quickly disseminated within companies.
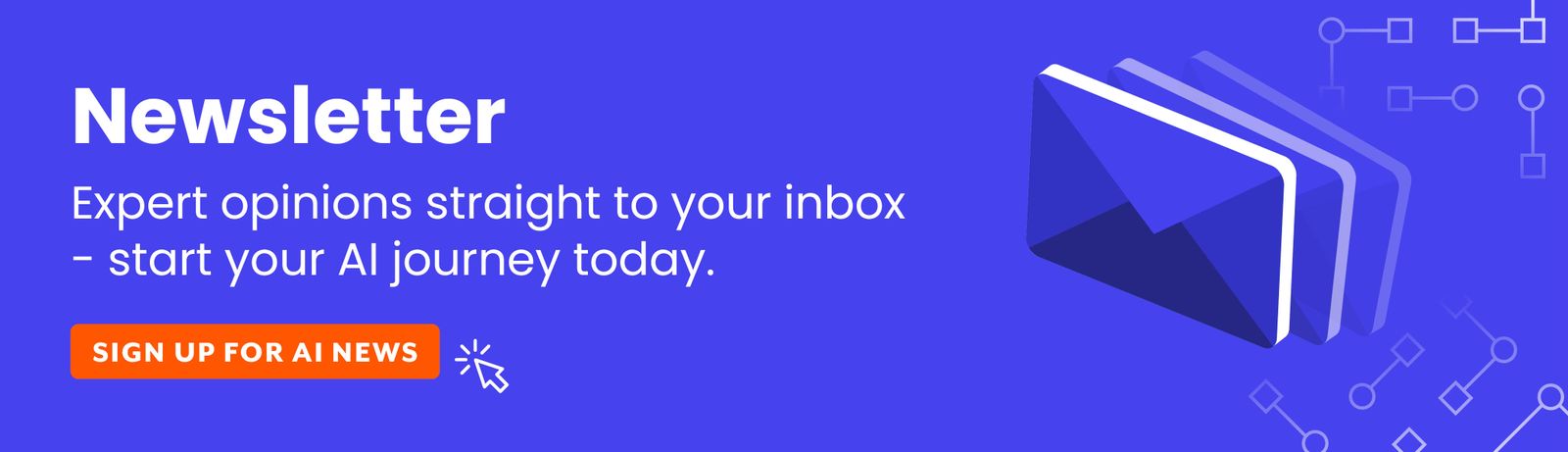
3. Demand forecasting
In the field of predictive analytics, demand forecasting estimates the forecast of customer demand through the analysis of historical data. This allows companies to avoid inefficiencies that can occur from supply and demand misalignment across operations. There are six common types of demand forecasting:
- External. For accurate external projections, like goals, growth, and competitors.
- Internal. Gives a detailed view of financials like product demand, supply chain issues, and operations.
- Passive. Beneficial when there’s a good amount of historical data.
- Active. Beneficial for newer companies, to predict scaling timeframes or forecasting investment funding.
- Short-term. Usually for a period under 12 months, predicting product demand, sales, and other inventory-based needs.
- Long-term. Similar to short-term forecasts but utilized for longer timeframes, such as two to four years into the future, and can look into supply chains, investment capital, and logistical issues.
AI can enhance demand forecasting through solutions such as:
Improved efficiency
Companies can focus on strategic issues instead of having to manage supply chain problems and unexpected stock fluctuations.
Improved manpower planning
Departments such as HR can benefit from demand forecasting, helping to optimize costs, make efficient part/full-time employee trade-offs, and more.
Customer satisfaction increase
Demand forecasting helps to accurately predict the amount of stock needed, making sure customers can buy products when they want. This leads to increased customer satisfaction and brand loyalty.
Better accuracy over time
Machine learning makes better predictions and forecasts every time it accesses new data, which makes it smarter and more accurate over time.
Markdown and discount optimization
Having unsold stock costs businesses money, and demand forecasting helps to move these slow-selling items by predicting which discounts and markdowns will gain customer interest.
4. Fraud
Machine learning algorithms are trained on historical fraud patterns to recognize them in future transactions. They can process data in real-time to detect CEO fraud, false invoices, payment fraud, and much more. Keeping up with the large-scale demand and modern sophisticated fraud attempts makes AI an ideal choice.
AI can enhance fraud prevention through solutions such as:
Identity theft
Whether it’s email addresses, names, passwords, bank details, or other information, identity theft is a major concern for both service providers and users. When there’s an account takeover, information is used to gain access to current accounts and details like email addresses can often be changed. With synthetic theft, true information and artificial details are merged to create a new personality for making counterfeit accounts and illegal purchases.
ID document forgery
Fake ID documents place automated verification systems, users, and service owners at risk. Machine learning can help prevent this type of fraud by scanning ID photos with a pre-trained neural network. The fraud detection system will then look for patterns present in other fake documents it was trained on, classifying the forgery and highlighting the need for further verification.
Payment fraud detection
The most common fraud type that AI tackles, payment fraud can include card ID theft, lost cards, stolen cards, and counterfeit cards. Loan scams or illegitimate charges are big concerns, with confidential information stolen (often by phishing) and used to order goods or request large loans from banks.
Email phishing
Confidential data is stolen by spreading fake messages and sites to users through fake access windows. With some messages looking legitimate, it can be difficult to distinguish a fraudulent email from a real one. Authentication protection includes factors like email verification, and network-level protection has three types of filters - blacklist, whitelist, and pattern matching. These work by banning IP addresses and domains from networks.
Fake account verification
Fake account detection is based on false activity and the rate of engagement. In social media, for example, fake accounts typically have a large number of followers or friends while the profiles have minimal user interactions. Features like the amount spent on the website, date of registration, MAC, and IP addresses of users’ devices can be used to identify users registering numerous inactive accounts.
5. Image and video recognition
Machine learning uses dedicated algorithms to extrapolate insights from hidden knowledge from a dataset of both good and bad samples, or supervised learning. Deep learning is the most common method, as it allows for expert real-time object detection and face recognition. Image recognition systems involve datasets with training data, the training of neural networks for image recognition, and AI model testing.
The processing of digital images includes the following phases:
- Image acquisition. Image capture with a sensor, which can be a camera, and conversion into a manageable entity. Can often be done by scraping.
- Image enhancement. Improves image quality to extract hidden information, allowing for further processing.
- Image restoration. Improves image quality by removing any possible corruptions, resulting in a cleaner version of the image.
- Color image processing. Processes colored images and different color spaces, which can be pseudocolor processing (colors assigned as grayscale values) or RGB processing (images acquired with a full-color sensor).
- Image compression and decompression. Compression lets image size and resolution be changed and decompression restores it to the original size and resolution.
- Morphological processing. Describes structures and shapes of objects within an image. Often used to describe what the AI model needs to recognize or detect.
- Image recognition. Identifies specific features of specific objects within an image.
AI can enhance image and video recognition through solutions such as:
Edge detection
Detects discontinuities in brightness to find edges of objects in processed images.
Object detection
The categorization of various different objects in an image, highlighting the location of each object through bounding boxes.
Instance segmentation
Differentiates various objects, or instances, that belong to the same class.
Object segmentation
Identification of specific pixels that belong to each object in an image instead of drawing bounding boxes around separate objects.
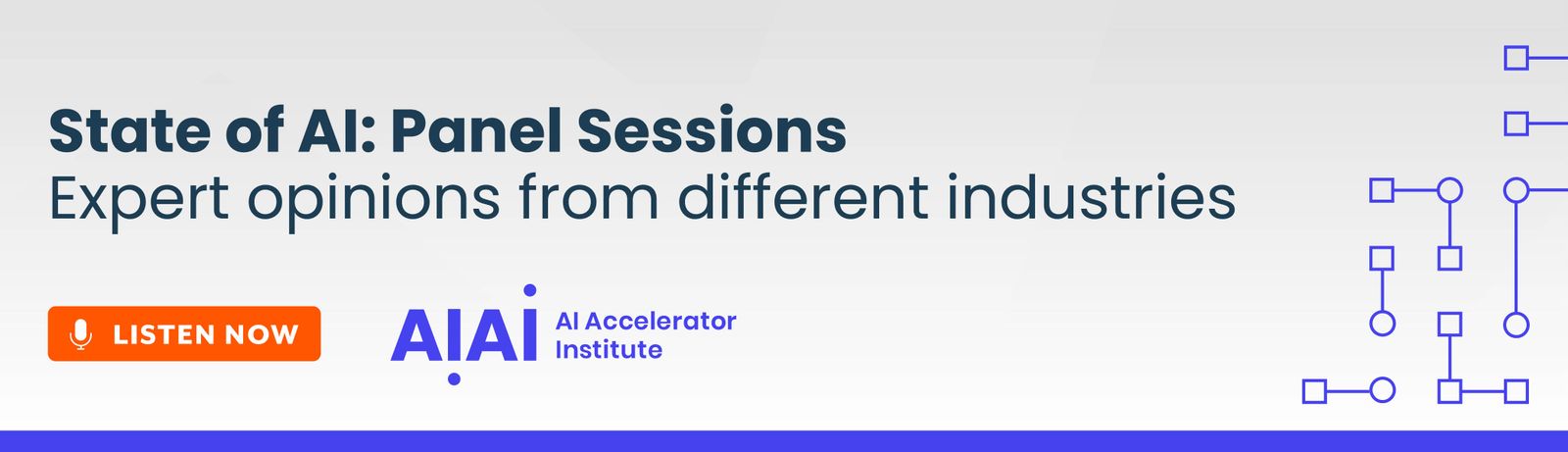
6. Predicting customer behavior
AI is having a big impact on customer behavior prediction, especially in the retail industry, as it’s helping businesses to make data-driven decisions. Leading to improvements across eCommerce, automation, manufacturing, supply chain, and more, AI also allows for personalized customer recommendations based on likes and dislikes, frequently purchased items, and more.
Customer behavior prediction involves:
- Segmentation. Customers are separated into smaller groups based on their purchasing behaviors, helping to identify regions in the market.
- Predictive analytics. Statistical techniques analyze historical data in order to predict customers’ future behavior.
AI can enhance customer behavior prediction through solutions such as:
Effective marketing campaigns
With online data of online searches, past reviews, and view numbers, AI can help to create an effective marketing campaign by determining what receives the most engagement from customers. Marketers can then choose the right medium for their messages in order to generate more sales.
Enhanced customer communication
Chatbots help to automate FAQs and lead customers to the information they’re looking for. They allow for quicker communication with customers, delivering higher satisfaction. As responding to each message can be challenging for bigger businesses, AI-powered chatbots free human employee resources for other business operations while engaging with customers in real-time.
Trend prediction
AI can mine data from past sales and reviews, even information from social media websites, to know what customers expect or what goods are popular. Also taking economic conditions and spending power into account, AI tools predict future demand and supply with extremely high accuracy. This allows companies to deliver personalized goods and services in targeted market regions.
Customer sentiment analysis
This helps companies to discover customer emotions - positive, negative, or neutral. By analyzing thousands of online product reviews, AI can pinpoint the level of customer happiness with price and quality. Companies can then make decisions and take action about product affordability and quality, improving customer service.
Customer churn prediction
Also known as customer turnover, customer churn relates to the percentage of customers who stop doing business with a company. Identifying the cause of customer churn and predicting it can help businesses take proactive steps and understand behavioral patterns.
7. Productivity
AI is making big changes in the manufacturing industry, for example, thanks to innovations in big data storage and analysis and cloud computing. AI systems offer critical information for companies to make data-driven decisions and improve their operations.
AI can enhance productivity through solutions such as:
Predictive maintenance
By using sensors that track equipment conditions and analyze data on an ongoing basis, companies can service equipment when it needs, instead of scheduling maintenance times. This leads to reduced downtime, as machines can also evaluate when they need to be repaired and order replacement parts as needed.
Automated material procurement
Machine learning and analytics record all kinds of data, including quoting and supply chain information. By automating material procurement, companies could reduce warehousing and transportation costs, as AI could help to make strategic decisions in sourcing and procurement.
Accurate demand forecasting
AI and machine learning can test hundreds of models, becoming more accurate while adapting to new data. Something as simple as taking physical inventory can be made more efficient, as automated machines like drones can keep track of items in real-time.
Manufacturing process optimization
AI systems can monitor cycle times, used quantities, lead times, temperatures, errors, and downtime to make sure production runs optimally. With AI running in the background, operators can receive suggestions and react in real-time, with AI learning from operators’ final decisions. This will allow for intelligent automation that will increase production efficiency.
Regulatory compliance
Various industries face rigorous compliances to follow. With these being constantly moving targets, AI and machine learning can help companies to navigate changes in regulations. These tools can also ease compliance processes and make sure that businesses don’t face heavy fines for not meeting important regulations.
Curious why more companies aren't adopting generative AI? Read more about it below:
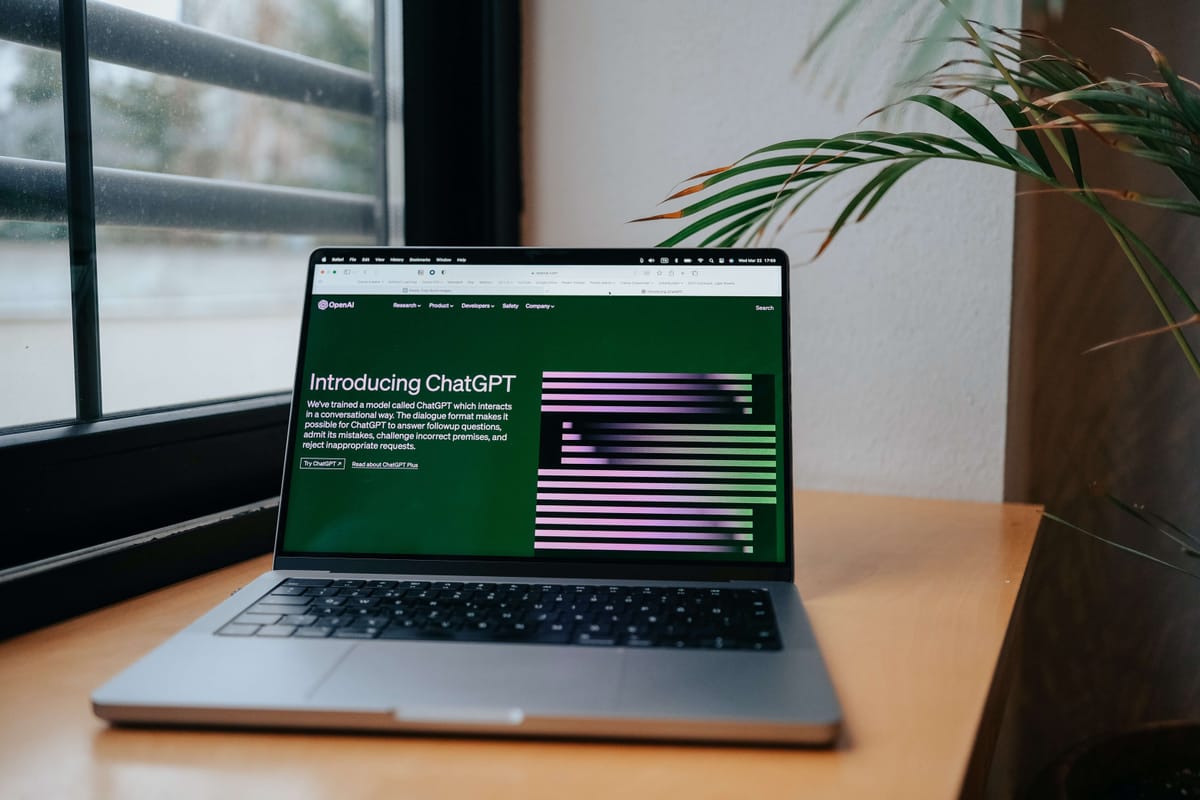