Artificial intelligence (AI) is being discussed nearly everywhere these days, including in legal circles. AI promises efficiency and objectivity, which are sorely needed in the justice system, but there are also horror stories, including racial bias against criminal defendants and even innocent individuals being wrongfully arrested.
The root cause often lies in the inherent biases within some algorithms that power AI systems, but the problem runs deeper than that. It's also about the data the systems are trained on, the goals we set for AI systems, how those are applied, and how we interpret the results. It’s not just technology - it’s us.
Enter Public Interest Technology (PIT), which we can think of as an essential mindset that focuses us on selecting, implementing, and evaluating AI systems in ways that are fair, just, and human-centered. It’s an approach that sets our sights squarely on the decisions that are most important when it comes to protecting people from the actual harms of bias and discrimination.
Public Interest Technology can act as a guiding framework that supports the development, implementation, and governance of AI in the criminal justice system to ensure fairness, transparency, and accountability.
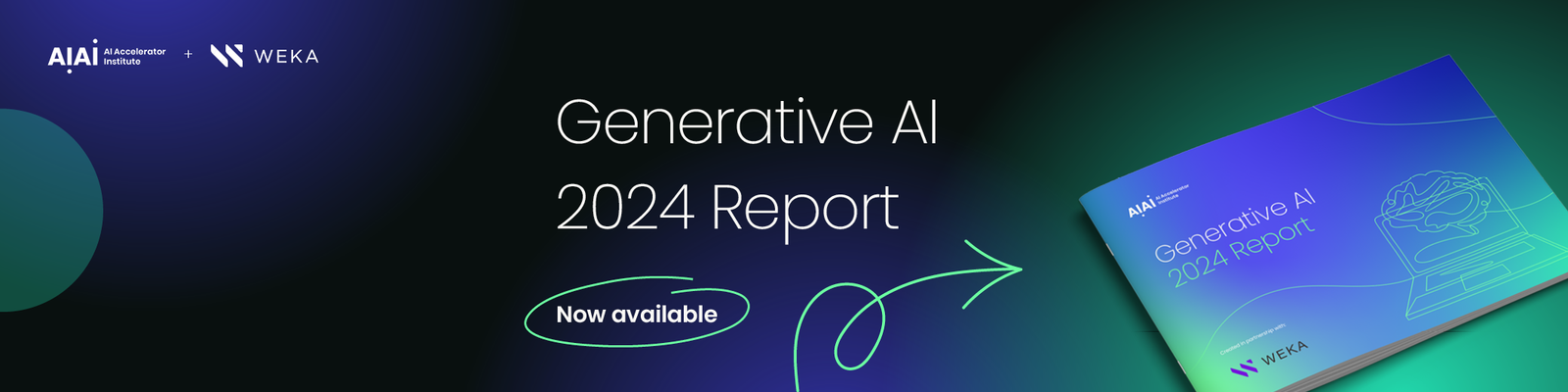
What exactly is Public Interest Technology?
Public Interest Technology is a human-centered approach to technology that prioritizes social justice, fairness, and equity in the design, development, and implementation of technological solutions.
Darren Walker, president of the Ford Foundation, explains that PIT focuses less on the technology itself and more on ethics, human rights, and social justice [1]. It emphasizes a socio-technological approach that prioritizes people's needs over unchecked technological development. In essence, PIT seeks to ensure that technology serves us and not the other way around.
This means designing, using, and regulating technology to benefit everyone, especially those from vulnerable or historically marginalized groups. It's about making sure everyone has a say in decisions about the tech that affects their lives.
AI in justice contexts
AI is already used in the criminal justice system to identify suspects, predict re-offense risk, and suggest criminal sentences. These are all powerful tools that promise to improve justice outcomes and positively affect society as a whole.
However, these same tools can and have perpetuated discrimination when not carefully and thoughtfully applied.
According to the ACLU, “...there have been at least seven wrongful arrests we know of in the United States due to police reliance on incorrect face recognition results — and those are just the known cases. In nearly every one of those instances, the person wrongfully arrested was Black” [2].
Further, recidivism prediction tools, such as COMPAS, have been criticized as unfairly categorizing Black men as high-risk for reoffense when compared to their White counterparts [3]. Some criminal courts are using this information to inform the sentencing decisions judges make [4]. Even worse, these AI tools are often opaque, meaning the decision-making processes they use are either unclear or entirely unknown.
Tackling algorithmic bias head-on
Algorithmic bias in facial recognition and recidivism prediction tools occurs in part due to biased data, poorly devised algorithms, and problematic feature sets. But it’s also due to a lack of human guidance and governance structures that restrain, shape, and guide the safe implementation of the technology. PIT not only emphasizes improving the technology itself but also stresses continued human management of those systems to recognize, address, and eliminate biased outcomes altogether.
For instance, researchers in New Zealand are developing transparent models for assessing assault cases in criminal courts [5]. Unlike the COMPAS program described above, these researchers are developing transparent AI models that open the model's decisions to scrutiny. By making the inner workings of the AI clear, it's easier to identify and correct potential biases and thereby prevent harm.
This aligns with the core PIT principles of transparency and accountability that contribute to fair outcomes and societal trust in these systems.
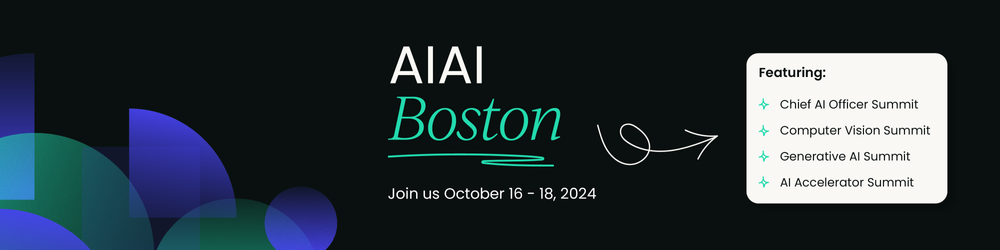
Human in the Loop
In addition to improving transparency, PIT also highlights the importance of human oversight. The concept of having a human in the loop is mandatory to ensure fairness, accountability, and transparency [6]. AI can be powerful in many respects, but it cannot replace human judgment, especially in high-stakes settings like the justice system.
Humans should not only be involved in developing and using AI, but they should always have the power to override AI-based decisions in any given case. This doesn’t guarantee fairer outcomes (human judges can be biased, too), but it does create accountability for the final result. It’s impossible to hold an algorithm accountable. It’s entirely possible to criticize and potentially remove an unfair judge.
A fairer tech future
PIT isn't a magic solution. Mindsets alone will not solve the problems that AI poses to society. However, it does focus our attention on implementing AI systems in ways that promote justice and equity, especially in the most sensitive of areas, like the criminal justice system.
By upholding values like fairness, transparency, and human oversight, PIT can help us minimize AI risks and ensure that this powerful technology serves society as a whole.
As AI becomes further intertwined with our lives, PIT will become even more crucial. By working together – technologists, policymakers, advocates, and the public – we can build a future where AI is a force for good, not harm.
After all, technology should always be a tool for justice, not a weapon of discrimination.
References
[1] Walker, D. (n.d.). Deprogramming Implicit Bias: The Case for Public Interest Technology. https://doi.org/10.1162/daed_a_02059
[2] Wessler, N. F. (2024, April 30). Police Say a Simple Warning Will Prevent Face Recognition Wrongful Arrests. That’s Just Not True. | ACLU. American Civil Liberties Union. https://www.aclu.org/news/privacy-technology/police-say-a-simple-warning-will-prevent-face-recognition-wrongful-arrests-thats-just-not-true#:~:text=To%20date%2C%20there%20have%20ben
[3] Angwin, J., Larson, J., Mattu, S., & Kirchner, L. (2016, May 23). Machine Bias. ProPublica. https://www.propublica.org/article/machine-bias-risk-assessments-in-criminal-sentencing
[4] Hao, K. (2019, January 21). AI Is Sending People to Jail—and Getting It Wrong. MIT Technology Review. https://www.technologyreview.com/2019/01/21/137783/algorithms-criminal-justice-ai/
[5] Rodger, H., Lensen, A., & Betkier, M. (2022). Explainable artificial intelligence for assault sentence prediction in New Zealand. Journal of the Royal Society of New Zealand, 53(1), 133–147. https://doi.org/10.1080/03036758.2022.2114506
[6] Mosqueira-Rey, E., Hernández-Pereira, E., Alonso-Ríos, D., Bobes-Bascarán, J., & Fernández-Leal, Á. (2022). Human-in-the-loop machine learning: a state of the art. Artificial Intelligence Review, 56. https://doi.org/10.1007/s10462-022-10246-w
Interested to know more about bias and the human mind?
Make sure to give the article below a read: