What do we mean by “Ethics” in AI?
Ethics involves the broader considerations of artificial intelligence (AI) and how it plays a role in society beyond the code. With Data Science holistically being a working capital-hungry, high-risk investment, ensuring AI is deployed without denigrating the rights and freedoms of individuals is key. So, let’s start with a big one that often is brought up: automation.
Ethical considerations: Automation
At the very heart, AI is a tool. Yes, it’s an incredibly fancy one but it’s a tool. One of the key areas where AI ethics comes into play is automation. Recently I’ve written an article “Is Artificial Intelligence killing creativity?” which’ll also cover some of my thoughts on this area.
If we take the Arts sector, for example, some fear AI will take jobs people have trained for years on: a very valid concern, and it’ll only be a matter of time to see how this pans out.
According to the World Economic Forum Future of Jobs Report 2023, example jobs likely to see the largest decline are clerical and secretarial roles. In addition, an Accenture paper “A new era of generative AI for everyone” predicts approximately 40% of all working hours could be impacted by Large Language Models (LLMs) such as ChatGPT- 4 (Chat Pre Trained Generative Transformer).
This is as a result of those language tasks accounting for 62% of the total time employees work. A key consideration to keep in mind though is machines won’t just replace humans: through a combination of augmentation and automation, 65% of the time spent on language tasks could be transformed into productivity.
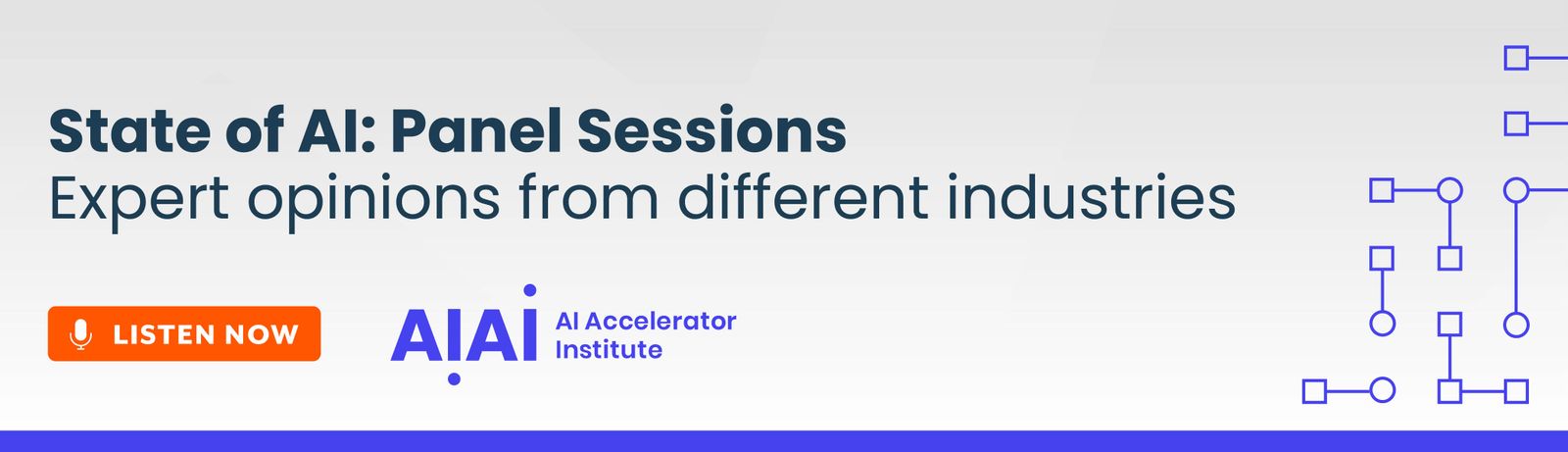
Ethical considerations: Bias
We then turn to another key ethical consideration. Bias, whilst not being new, is one of a number of areas to consider with AI systems. I think of bias as an entity wrapped in an invisibility cloak: it can easily intertwine its way through training data (the data we train the system to make decisions on), even if special category data (ie: gender, race) is removed.
There’s plenty of research going into bias in AI systems, and as a result, the issue of bias creates an interesting domino effect of how it can occur in a cycle of human decision making which could then seep into system design.
With the above in mind, within bias comes the concept of fairness. Now this is where things get even more intriguing. Measuring fairness in itself can create barriers. Technical ways of defining fairness have been created, for example, the requirement of equal predictive value.
In addition, another is to require models to have an equal false positive and false negatives across groups. It’s important to note thought that differing definitions of fairness can’t always be simultaneously satisfied.
In summary, mitigating bias isn’t just about tuning or changing algorithms. Let’s think about human-in-the-loop systems: having humans and machines working together is a powerful combination.
Humans being able to select options generated by the system allows for a holistic assessment of how much weighting should be given to a system-generated recommendation, thus in the longer term being useful in increasing both confidence and transparency.
Ethical considerations: Privacy
The last part I’ll cover is in my view the backbone of ethics: privacy. This is an area that can result in a continued tug of war within organizations: respecting the customers' privacy versus using data to enhance their experience to keep them loyal.
The UK has some of the toughest privacy laws through the General Data Protection Regulation (GDPR). With petabytes of data being shared around the world every second, bringing AI into the mix creates murkier waters: a system could identify an individual who originally wasn’t identifiable from the input datasets standpoint.
On a separate note, whilst the input data into an AI system may be straightforward the data processing in the “black box” could still reveal unwelcome surprises. Whilst it’s impossible to eliminate the risk completely, completing a Data Protection Impact Assessment (DPIA) is crucial in both understanding and minimizing these risks.
As information sharing increases and AI-based systems become more advanced, AI and privacy will continue to be a long and complex road to navigate. As a result, regulating these systems to ensure they don’t get out of control will be key, and one to watch too.
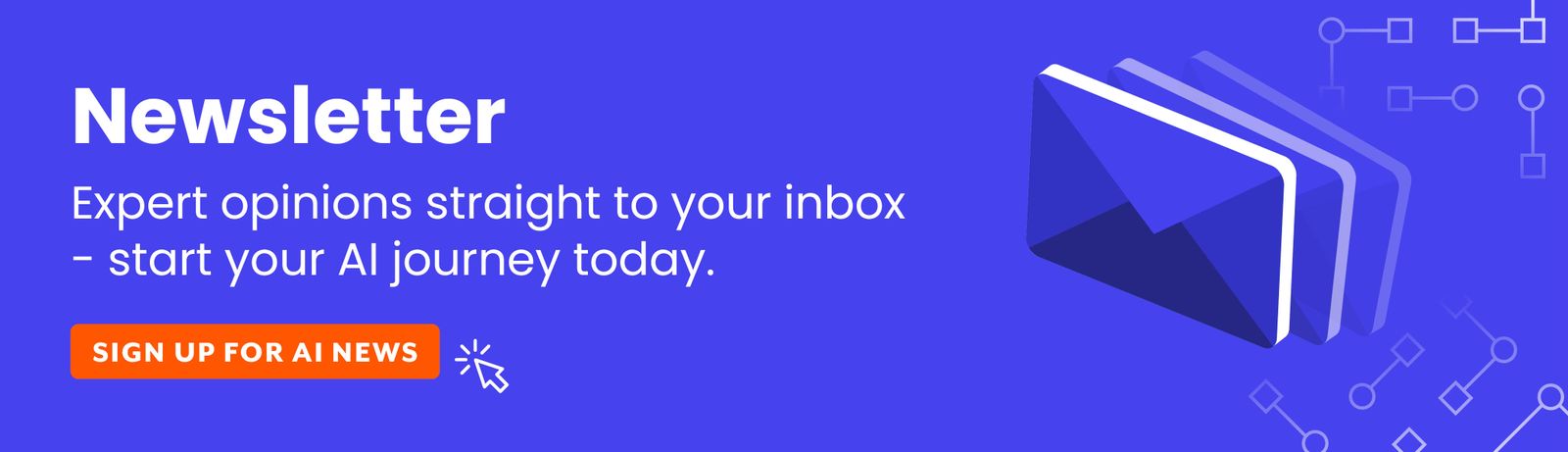
Conclusions
In summary, ethics is a fascinating part of a data journey. The rapid rise of ChatGPT has resulted in a paradigm shift in how we think about AI and ethics. Irrespective of whether you’re a startup or a multinational, I can’t emphasize this enough: put ethics at the heart of your data strategy: don’t just launch into the fancy code.
Bibliography
Gordon, C. (n.d.). 2023 Will Be The Year Of AI Ethics Legislation Acceleration. [online] Forbes. Available at: https://www.forbes.com/sites/cindygordon/2022/12/28/2023-will-be the-year-of-ai-ethics-legislation-acceleration/?sh=345d8fade855 [Accessed 12 May 2023].
Manyika, J., Silberg, J. and Presten, B. (2019). What Do We Do About the Biases in AI? [online] Harvard Business Review. Available at: https://hbr.org/2019/10/what-do-we-do about-the-biases-in-ai.
A new era of generative AI for everyone. (n.d.). Available at: https://www.accenture.com/ content/dam/accenture/final/accenture-com/document/Accenture-A-New-Era-of Generative-AI-for-Everyone.pdf.
Bossmann, J. (2016). Top 9 ethical issues in artificial intelligence. [online] World Economic Forum. Available at: https://www.weforum.org/agenda/2016/10/top-10-ethical-issues-in artificial-intelligence/.