Let's start with the basics: building an AI product is different from using AI to build a product. Consequently, the product manager for AI is not simply a PM that uses AI. They can use AI for sure but their primary focus is to build, deliver, and manage AI products for the end-users.
With that in mind, let's move to a second clarification: AI products have different stages compared to traditional products. However, they are still products and the PM must know where to be involved and how.
The product management lifecycle
Traditionally Product Managers manage the lifecycle of the products in a very specific way, which can be described in five steps:
- (Product) Ideation (Discover).
- Concept testing (Define/Validate).
- (Product) Development (Implement).
- Delivery (Launch).
- (Product) Retirement (Measure).
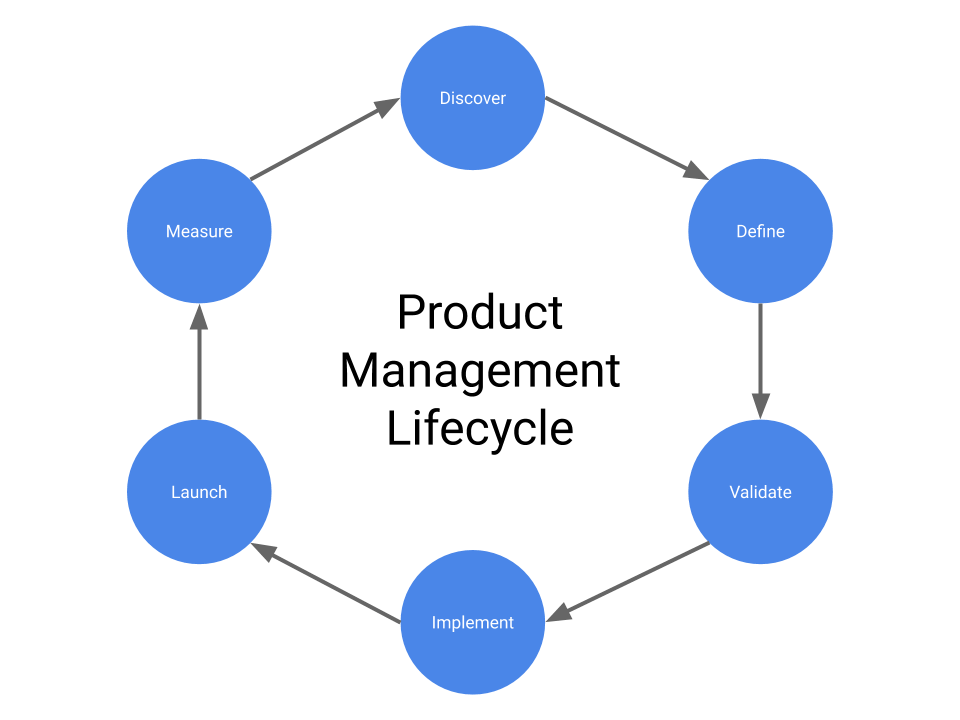
When it comes to AI products, an inner lifecycle is introduced and needs to be blended by the product manager into the above steps. Let's take a look at the machine-learning (ML) lifecycle compared to the product lifecycle.
The ML lifecycle
ML lifecycle may be defined as an iterative four-step cycle:
- Scoping.
- Experimentation.
- Training.
- Serving.
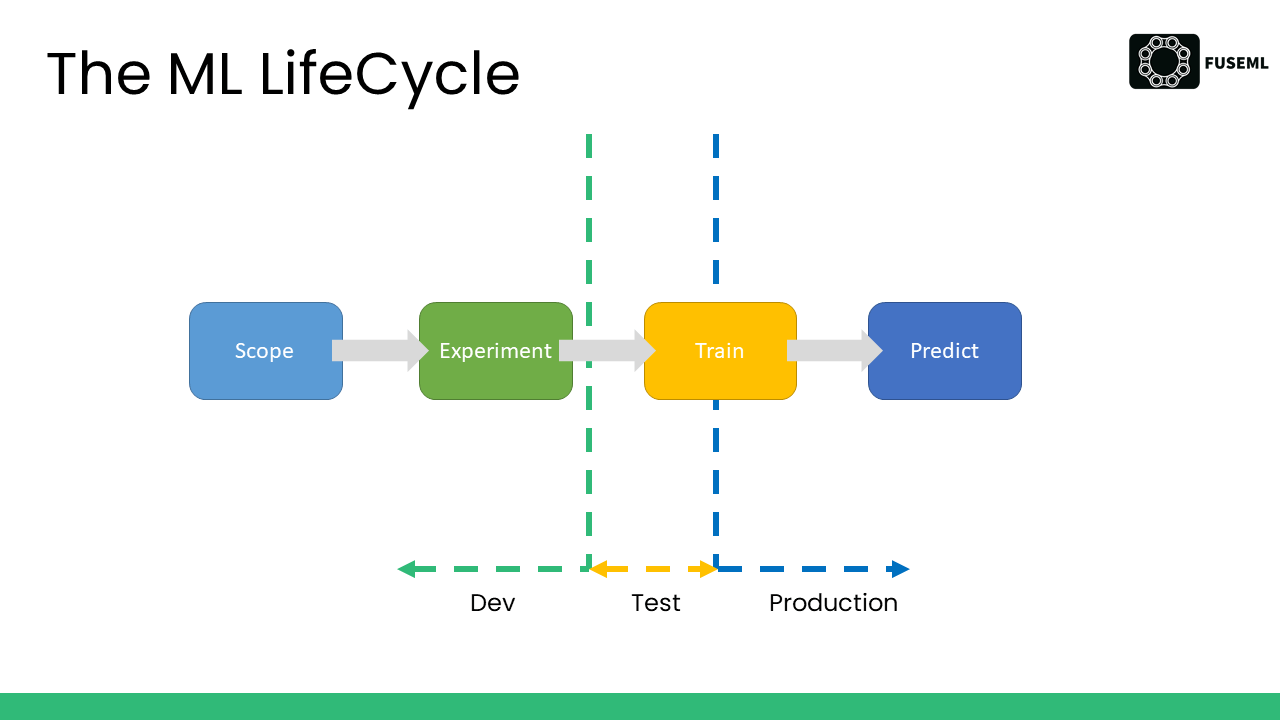
Here, we can briefly describe each of them:
Scoping
When a new AI product has been envisioned the team who are responsible for building it has to define the scope of the project itself. In this phase, the team has to answer specific questions, such as:
- What we are building?
- Where is the data we need to use?
- How can we ensure the data has the right quality before we use it?
In other words, it’s about understanding the problem and the scope of effort involved in each component of the team, in each phase of the project.
Experimentation
In this phase, the focus is on producing a "prototype" of the product, which means we either focus on writing and selecting the right approach (batch of data vs streaming of data) or which mathematical model seems to give back the best outcomes?
This is an unpredictable phase because it may require a long time for the data scientist and the data engineer to find the right solution.
Training
The training part may not be part of your journey, but in the majority of cases a pre-trained model is going to be used. If you’re building an NLP (Natural Language Processing) solution, a.k.a a digital assistant, chances are that you’re going to use something like GPT3 as an underline model.
In all other cases, the training part will occur, and this will give us an idea of how much the model performs, if we need to come back to the experimentation phase to refine our approach, or if we may move toward the production phase (serving).
Serving (Predict)
This is the last part of our AI journey. Once the model has been trained we have to "pass" it to the inference engine where it will be tested against real data. In other words, the AI product we built will make use of the model against real data queries (i.e our digital assistant starts to answer end-user questions).
At this point, our journey is just at the beginning. The model will lose accuracy or precision over time, in other words, drifts will occur either in terms of data drifts or content drifts. Our team will have to come back to previous phases: re-train the model, apply a new pre-trained model, change the approach and develop a completely new strategy.
This iterative process is never-ending since our data and the way we end-use the product will change over time and we have to adapt our product or retire it.
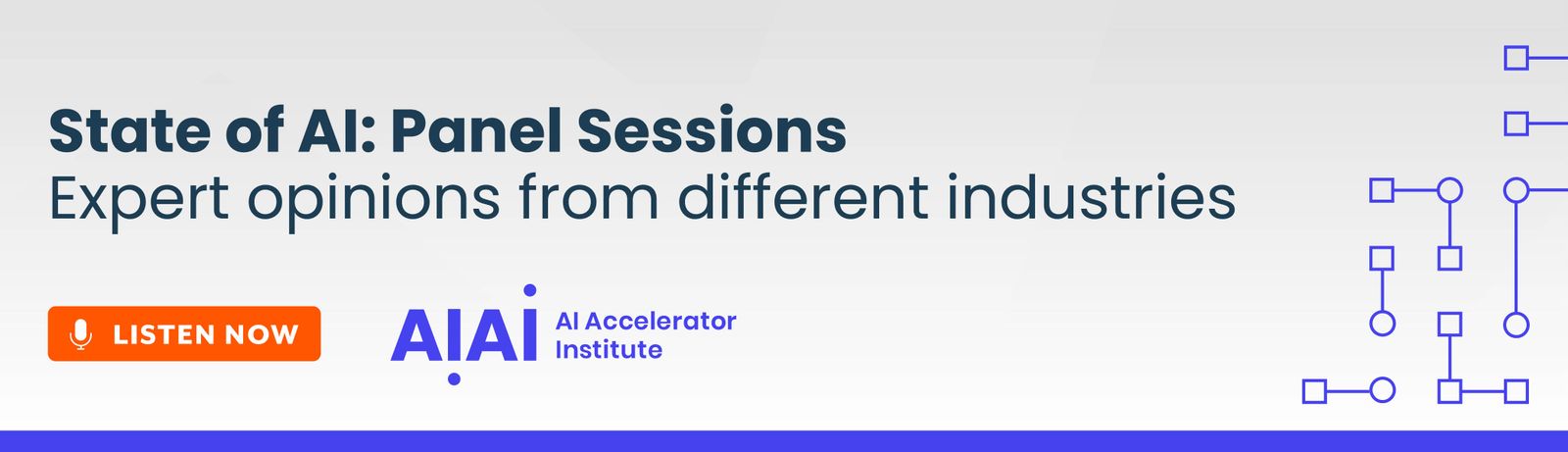
Fit the PM role into the AI project
So where should the PM be involved and how?
Let's compare the product phases with the ML lifecycle phase:
- Scoping vs. ideation.
- Experimentation vs. A/B testing.
- Training vs. development.
- Serving vs. delivery.
- Iteration of the process vs. "retire or evolve" phase.
Scoping vs. ideation
I hear many stories where it seems that the scoping of an AI product/project is an "engineer" only task. Nothing could more wrong.
While the engineering team is responsible for understanding the technical details, those need to be fitted into the product ideation. The questions the team (including the PM) should answer first are:
- Why we are building this product?
- Why should the end-user use it?
The PM has the responsibility to drive the vision, so always be sure that the team has a clear direction. Keep in mind, it's still a product and will be used by end-users so never underestimate usability, clearness of vision, and continuous improvements.
Experimentation vs. A/B testing
The team will spend quite some time understanding the right approach: data quality, mathematical approach, etc. This time cannot be more useful to you as a product manager to do some quick prototypes and run some A/B testing.
It's all about understanding the final product and cutting off the time to get a greater sense of what your AI product will look like.
Training vs. development
Our job as a product manager should be to delight customers with an amazing product, no matter if those customers are our internal users or external ones. We have to delight all of them through fast delivery, continuous improvements, and attention to their needs.
The training phase may require a lot of time, due to the technicalities of the process itself, but it's a perfect moment where we may prioritize other activities.
The model can be chosen and consequently we’ll be able to serve it to the "prediction engine". We can also use the time to work further on the final application architecture and work out the release plan.
Serving vs. delivery
Finally, the model has been trained, or maybe you skipped that phase and used a pre-trained model. The application may start to use the model and make the right predictions. This is when your job as a PM starts for real.
The moment the model lands on the prediction service is the moment you’re ready to execute your launch plan. This is when your early adopters may have a glimpse of the product and you’ll start to collect data to refine the strategy. The faster you land on this phase the earlier you'll start to refine your trajectory toward a successful launch.
Iteration of the process vs. "retire or evolve" phase
I explained above how the ML lifecycle is an iterative process and the model has to be re-deployed over time, while this practice may induce the PM to think the product is improving, this is a false perception.
The capability of your product to be improved in terms of technical capabilities (predictions) has nothing to do with how the product is perceived by the end-users. You are responsible to check if you’re still answering the right questions: Why we built this product? What problem is it solving? Why should the user choose us?
Conclusion
To conclude the work of an AI product manager is challenging due to the nature of an AI project but it can still fit the usual process of product management. We have to keep that in mind and avoid being dragged into a "technical exercise" just to be able to say "we did an AI product."