This article comes from Dr Nikolay Burlutskiy’s talk at our London 2024 Generative AI Summit. Check out his full presentation and the wealth of OnDemand resources waiting for you.
Nikolay was the former Director of ML and AI at AstraZeneca at the time of this talk. He's currently the Senior Manager of GenAI Platforms at Mars.
Have you ever wondered just how long it takes to bring a new medicine to market? For those of us working in the pharmaceutical industry, the answer is clear: it can take decades and cost billions. As a computer scientist leading the AI team at AstraZeneca, I've seen firsthand just how complicated the drug discovery process is.
Trust me, there’s a lot of work that goes into developing a drug. But the real challenge lies in making the process more efficient and accessible, which is where generative AI comes in.
In this article, I’ll walk you through the critical role that AI plays in accelerating drug discovery, particularly in areas like medical imaging, predicting patient outcomes, and creating synthetic data to address data scarcity. While AI has incredible potential, it’s not without its challenges. From building trust with pathologists to navigating regulatory requirements, there’s a lot to consider.
So, let’s dive into how AI is reshaping the way we approach drug discovery and development – and why it matters to the future of healthcare.
Generative AI’s role in enhancing medical imaging
One of the most powerful applications of generative AI in drug development is its ability to analyze medical images – a process that’s essential for diagnosing diseases like cancer, which can be difficult to detect early on.
In the world of pathology, we’re increasingly moving away from using traditional microscopes, and using digital images instead. With digitized tissue biopsies, we now have access to incredibly detailed scans that show every single cell. The sheer volume of this data – sometimes containing over 100,000 x 100,000 pixels – makes it almost impossible for human pathologists to analyze every single detail, but AI can handle this level of complexity.
At AstraZeneca, we’ve been using generative AI to help analyze these vast datasets. One of the most exciting developments is in medical imaging, where AI models can quickly identify cancerous cells, segment different areas of tissue, and even predict patient outcomes.
For example, AI can help predict whether a patient will respond to a specific drug – information that’s invaluable for companies like ours, as we work to develop treatments that will provide real, tangible benefits for patients.
In my work, we leverage powerful AI techniques such as variational autoencoders and generative adversarial networks (GANs) to build these models. These AI techniques can help us learn from medical images and generate synthetic data that can be used to train AI models more effectively.
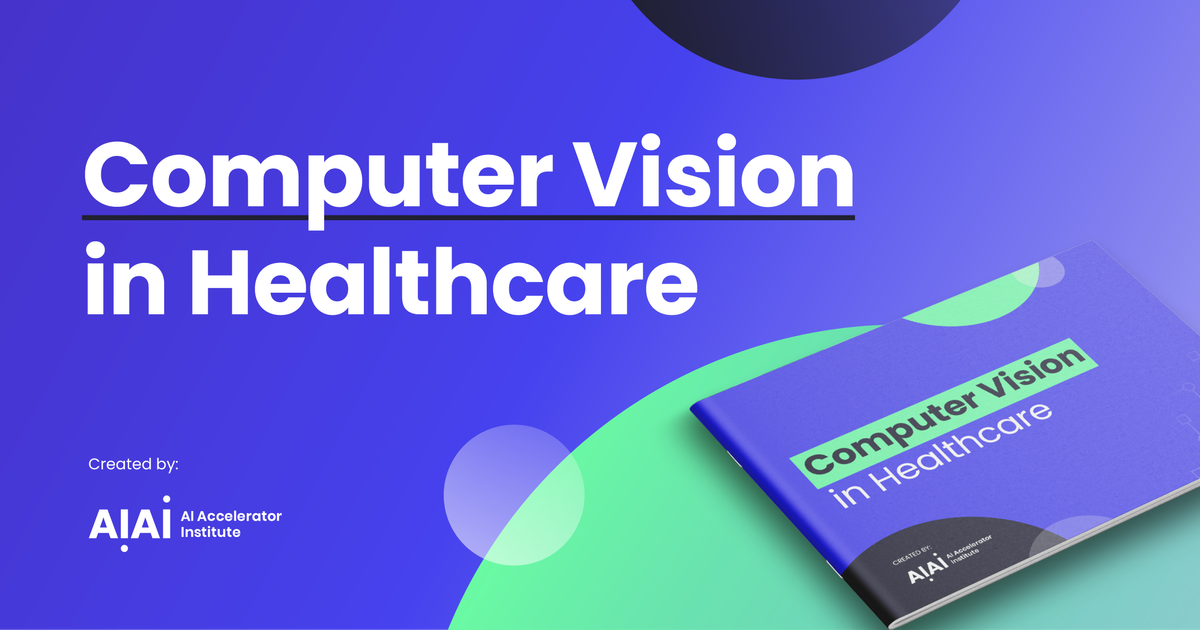