For those that don't know, Qualcomm has been around for 36 years. It was founded by Irwin Jacobs and six others in San Diego, where we’re still headquartered today.
Right from the start in 1985 through to today, our focus has been on connectivity. And although the underlying technology has changed, what hasn't changed is our overall strategy and focus around investing in technologies that enable worldwide connection. Our efforts around artificial intelligence (AI) are well aligned to the initial vision our founders shared.
At Qualcomm, we invent breakthrough technologies that transform how the world connects, computes, and communicates. Initially, we led the mobile industry into the digital age. Then we connected smartphones to the internet and essentially put mobile computers in our pockets, unlocking whole new capabilities and industries.
Today, we're really taking 5G to virtually every other industry, which I'll touch upon today in my talk, which is focused on AI and computer vision.
By leveraging this mobile technology, we’re enabling new experiences or what we call ‘distributed intelligence.’
Essentially, distributed intelligence is marrying the fast and super secure mobile AI processing on your device, and connecting that with the powerful AI capabilities of the cloud through high-speed, ultra-low latency 5G connection, enabling new use cases that we've never seen before.
Through this 5G connectivity and leveraging our mobile DNA or low-power processing DNA as well, we're bringing cloud and the edge closer to the consumers, delivering new user experiences, and seamlessly working with our industry partners.
Qualcomm has been working on AI for over two decades. I know it might seem quite wild, but this was before AI was as exciting and popular as it is today. But we've been thought leaders in this space for many generations, and we’ve had successful AI commercial products in the market for many years as well.
We've historically been focused on the mobile space from a facial recognition, voice assistance, and real-time translation perspective, with over six generations of AI-enabled devices in the market.
With the Cloud AI 100, we're leveraging much of that knowledge into what we're doing today on the cloud and edge markets, focusing on low-power processing and AI.
Qualcomm’s solutions optimize AI performance
So what does this all mean? The chart below demonstrates how we've addressed this amazing opportunity with AI from the sub-30 TOPS perspective, (trillion operations per second).
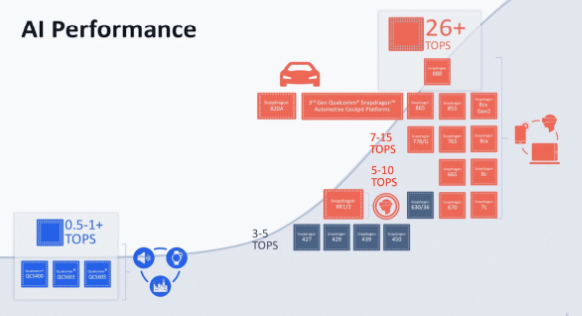
On the lower side of this chart in the bottom left corner is what we call our QSC product line. This addresses opportunities such as smart speakers, cameras, and sensors, leveraging our digital signal processing or DSP technology.
As you move up to the right side, we scale from three TOPS to 30 TOPS. You're getting more into opportunities from classic mobile, what we call Snapdragon, XR which is AR and VR, automobile offerings, which has been a great success story to Qualcomm around in-vehicle infotainment, and Windows on Snapdragon, which is our effort into the PC space. So a lot of solutions that historically span many markets already.
So, how do we build from there?
With the introduction of the Cloud AI 100 back in April 2019, we're now enabling new opportunities on the high end of the AI inference market.
As you can see on the top end of the graph below, on the right-hand side we have a robust set of offerings across different use cases and the Cloud AI 100 side. We have three discrete offerings: a greater than 50 TOPS solution, a 200 TOPS solution, and a 400 TOPS solution.
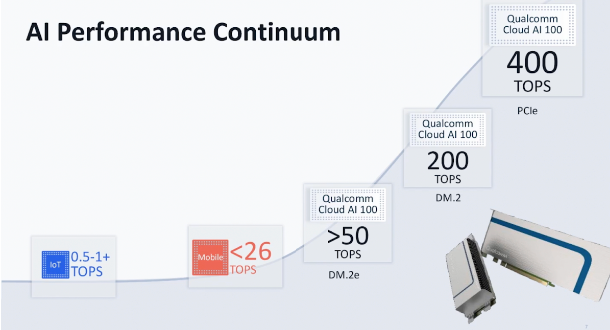
On the edge side, we've optimized what we call the Dual M.2e for this market. It addresses performance in the 50 TOPS space and above, at around 15W TDP. And we have a compelling form factor and low-power AI leadership that really makes it a great fit for applications like this.
The Dual M.2 is a standard OCP form factor and is optimized for the data center and hyperscaler. It's a best-in-class performance from this card and it’s targeted for data center applications.
And then up to the right of the graph, we have PCIe. This is your standard half-height, half-length, 75-wide card that addresses up to 400 TOPS with a thermal design and TDP perspective. These are really compelling solutions and are really scaling up our performance leadership around AI.
Seizing opportunities at the edge
Now, I’ll give you an idea of why we're excited about this space. This isn’t John's view of the world, this is an outsider's perspective on how big the market is. And externally, analysts are forecasting really explosive growth, especially in the AI inference market.
We're on the frontline seeing this. So we're working closely with customers and partners and bringing compelling solutions to market.
Below is a recent report from Omdia that shows explosive growth in AI, especially around inference, which is dominating the market today.
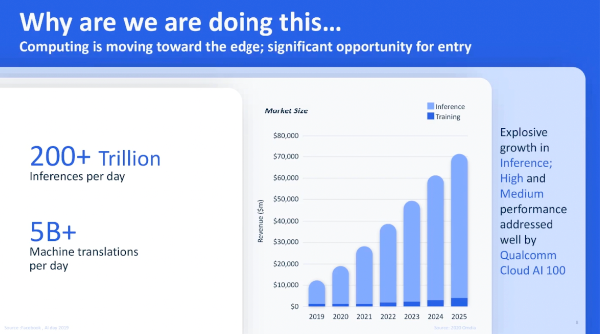
As we've seen in the past, the forecast is continuing to grow, and we're really excited to be at the forefront of this exciting growth.
This table is at a higher level and doesn't really align with what I talked about earlier around being greater than 50 TOPS. But if you peel it back, you’ll see the opportunity, especially in what they call the highest segment as being really where the growth is and where our Cloud AI 100 shines.
From a market landscape, we see a number of emerging opportunities that our family of AI accelerators addresses beyond even the traditional mobile, IoT, or PC. We’re really focused on three very interesting and compelling markets, and we're seeing very consistent and clear opportunities here.
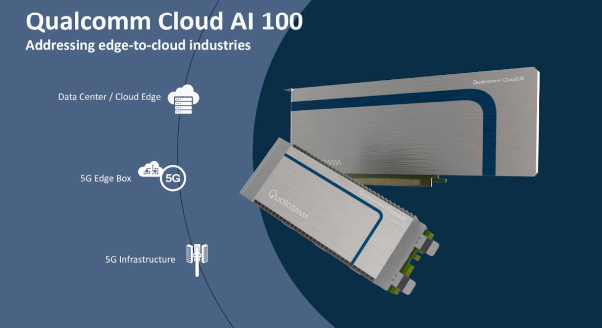
The market requirements are going to change from customer to customer for different solutions. I quickly touched upon the card types, and I'll get into that later, but we really view security, power, and software as a family of opportunities here.
First and foremost, on the data center/cloud edge, there are lots of cloud service providers, hyperscalers, opportunities around natural language processing (NLP), and recommendation-type networks.
With the 5G Edge Box, everyone's got a name for this; intelligent edge, edge gateways, or whatever it might be. With this on-premise embedded standalone device, we're seeing a lot of object detection and classification. The deployment could be light poles, enterprises, retail, etc.
This is a really compelling solution and opportunity we're seeing today, not only for our Cloud AI 100 but also for 5G technology and our AP technology as well.
And then on the 5G infrastructure side, this is an area that I'm particularly excited about. We're seeing a lot of classic equipment providers taking traditional computational intensive beamforming algorithms as an example, and replacing it with new neural net algorithms. So this is an area where we're helping to change the industry.
Let's dive into the product a little bit more.
Key features of the Qualcomm Cloud AI 100 cards
To deliver this industry-leading performance, we offer three different AI accelerator cards, from the standard half-height, half-length solution on the left-hand side of the chart below, which is mainly targeted at hyperscaler markets and the data center, to two different Dual M.2 edge cards that are aligned with OCP (Open Compute).
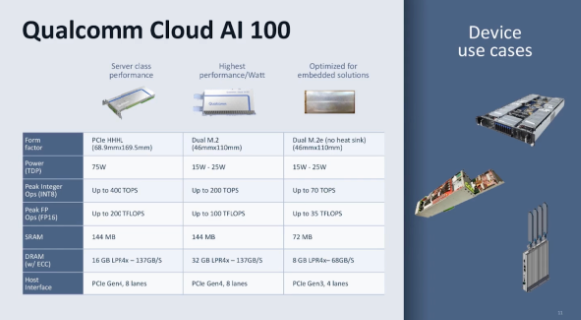
the Open Compute Dual M.2 solutions might be better suited for on-premise edge solutions like the one on the far right.
We configure each solution with the appropriate amounts of DRAM our customers request, and our Dual M.2 card is in the middle of our highest-performing TOPS per watt.
Each of these accelerators has a very high-level on-chip SRAM, approximately nine megabytes per AI hardware block. So each of these has different configures of embedded AI hardware blocks.
This allows us not to go out to the LPDDR quite as much, even though we use LPDDR4x in these cases. This helps drive higher performance per watt and lowers costs for our customers, rather than using other solutions like HBM or GDDR.
We do very high memory bandwidth when needed, and we can configure DRAM density solutions as well. Any of these cards go from eight gigabytes up to 32 gigabytes. The different configurations that are shown in each of the cards are driven by customer needs and requests that we see.
We're very focused on the performance needs of various card TDPs (thermal design power). We know that card TDPs drive many form factors and not the chip as well.
We also support PCIe Gen4 out to the host. These are offload engines and accelerators, so they pair up to a host processor. It could be a server CPU, mobile CPU, or whatever it might be. And so we support Gen4 by eight on our PCIe and Dual M.2 card.
And then on the far right side of the table, we did go down to four lanes of PCIe Gen3 for embedded solutions. We went to Gen3 to meet industrial requirements and temperature for outdoor deployments. We could also support Gen4 there as well, so we just optimized that solution.
We can support multi-card solutions, and we can improve performance with card-to-card communications through PCIe switch without going through the host solution for large networks. It could be recommendation networks as well.
These are the three key solutions that we’re driving today.
Qualcomm Cloud Edge AI 100 development kit
As I mentioned earlier, one of the really exciting opportunities that we see in the space is what we call the 5G Edge Box. I'll get into a little bit more detail about the solution that we've been providing since October last year. It's a development kit, but it gets customers started on this solution if they're interested.
We view the 5G Edge Box as a greenfield opportunity that we're really excited about and that can really benefit Qualcomm's comprehensive solution. Although the Cloud AI 100 is near and dear to my heart, we also have leading technology around AP application processors like the Snapdragon 865, and our 5G solution as well.
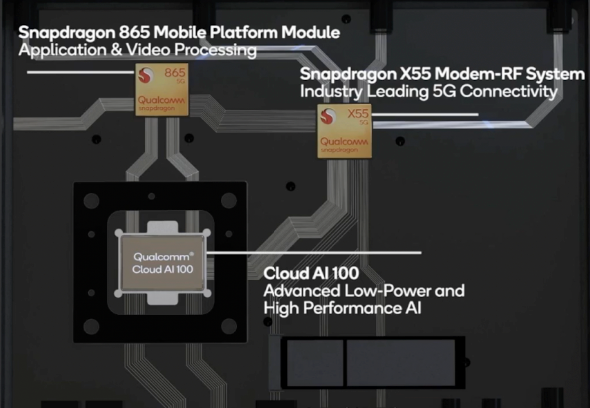
In order to drive this market, we introduced this platform last year. It's in the customer's hands, but it's really a development tool, specifically tailored and driven for Qualcomm to design and get customers started who are considering opportunities in this space. And we’re working with a number of partners to drive more turnkey commercial solutions in the future. So stay tuned on that front.
But this is a development kit that we've been sampling for about eight months now, and it’s a really compelling solution. Snapdragon 865 can decode 24 streams of full HD in a really thermal, stringent, mobile-like form factor, and 5G connection is there too.
Edge and data center use cases
I wanted to hit upon a couple of use cases. I could talk about this for days, but just in the interest of time, I’ve picked two different edge use cases and two different data center use cases. They're not meant to be comprehensive by any means, it's just meant to give you a little more insight into what we're seeing around Qualcomm for these experiences.
First, for the edge, this is what I think of as a classic type of retail use case. Maybe this is a small grocery store or pharmacy, where there's high-level AI and computer vision run on-premise.
This particular use case can help prevent loss and theft, and increase operational efficiency by understanding when customers are waiting and need support. It can also enhance safety through a classic image classification network, maybe a ResNet 50, identifying a bottle on the floor and alerting the store manager immediately to rectify the situation and potentially avoid injuries or a lawsuit.
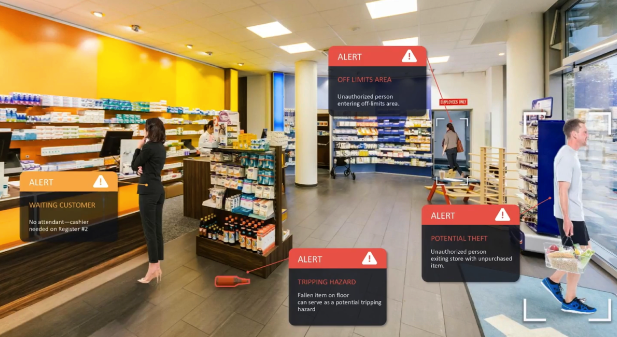
Here you have lots of different cameras capturing lots of different potential alerts in this particular case. This is a great example of where our Snapdragon 865, which has all of that video processing along with AI, comes into play. And maybe you have a 5G connection on box too. If a customer slips and falls, you might want to capture that image or video and store it in a larger data center for further insurance reasons.
Here's another example building off that. This is a use case you might all start to get reacquainted with. In the US, a lot of children are on summer vacation, and I see more and more that people are starting to travel again. So this might be a case that many can relate to.
Similar to the last example, this is another on-premise use case with various alerts for vision-based networks. This could be an object detection model, maybe putting a bounding box around an unattended suitcase. Or you could do object tracking, following the person running through the airport. That could be a safety hazard as well.
Or it could alert for an unattended child, understanding that there's no one around this child and they might need help and attention. I love to focus on those real positive aspects of AI and everyone’s safety.
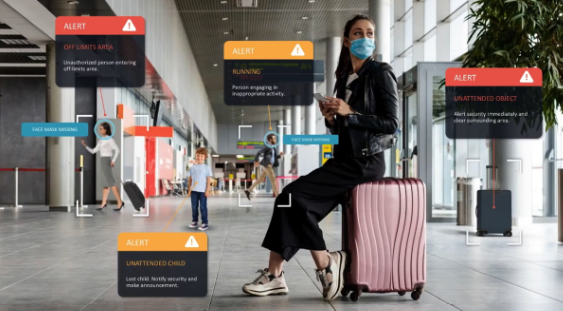
So those are some examples there, maybe transitioning a little bit more to cloud. The line kind of blurs from our perspective between the two, but I'll quickly touch upon this.
Focusing on social media, you see a number of exciting use cases from natural language processing. Maybe a model where you can converse with someone in real-time who doesn't speak your language, and it’ll feel very natural with no latency.
It could be recommendation networks on items you might be interested in based on past experiences. It could be visual from pictures you’ve made, or even past shopping experiences and really tailoring those.
Being a parent to teenagers myself, I'm especially interested in the inappropriate content area, whether it be videos or images that are captured and addressed through AI before getting posted. So that's something that's very important to me personally.
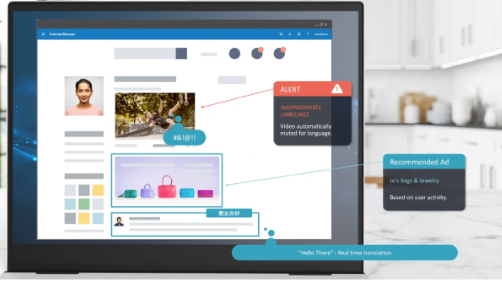
And this last use case is something I think we can all relate to as well, which is around medical and AI.
Below is an example that shows an x-ray and identifies a potential area of concern. Perhaps it makes a recommendation to medical staff based upon a trained model that might have been missed, that could impact lives and could affect safety and our health. So this is something that we're seeing a lot of really exciting use cases for as well.
Qualcomm Cloud AI 100 leads in performance per watt
I'll now touch on our solutions. You might be thinking, Hey, John, this is great. I understand your product, your use cases, and your markets. But why is your product compelling? Why is it interesting? Why should we pay attention to what Qualcomm’s doing?
We recently submitted our MLPerf numbers. We did two vision networks, a ResNet-50, and a ResNet-34 SSD submission.
Below was our first submission into MLPerf for the Cloud AI 100. This was just done for MLPerf 1.0, and we're excited to be involved in this moving forward with 1.1 coming up soon.
We submitted with models and form factors that we're essentially seeing strong customer interest and traction on. As I mentioned, ResNet-50 and ResNet-34 SSD are two that we submitted on. Not only did we submit two models, but we also submitted multiple form factors, form factors being those card types.
Above is a ResNet-50 example, which is a convolutional neural net focused on image classification. On the x-axis, as you go left to right, power actually goes down, and on the y-axis, as you go bottom to top, your performance goes up.
We’re really focused on performance and we compare it against industry-leading solutions in the market today, such as our friends at NVIDIA for example.
If we start on the x-axis, you can look at a half-height, half-length card with 70-75 watts against our solution versus a T4 solution, and you can see that we have approximately four times better performance at the equivalent power level.
T4 has been a great solution in the market, really industry-leading, so kudos to NVIDIA for doing a great job.
Next-generation solutions from NVIDIA are the A10 and A30. Those were recently announced at GTC, and they submitted those last cycle as well. The power did go up, they more than doubled the card TDP and it went to more of a full-height, full-length card. But as you can see, the performance for us with less than half the power is still industry-leading as well.
So, this is really looking at performance, in this case, ResNet-50. What they call offline performance is really peak performance, and that's a solution that we're really excited about. This is integer eight, for those that want to know all the details, and this is all public information as well.
We didn't include other solutions because they fall outside the scale of this chart. We try to compare apples to apples.
The next one we did below is a very similar setup. This is an SSD-ResNet 34. Similar to the ResNet-50, you look on the left-hand side and you really focus on the data center. On the right-hand side, these are edge as well.
I should note that on the left-hand side, when we did a power submission for MLPerf, I compared performance with publicly noted card TDP numbers. So these aren’t necessarily power submissions, but I just normalized it for the card TDP number.
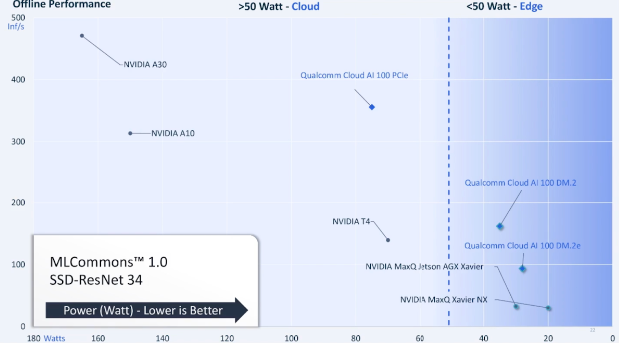
This also doesn't capture great things like batch sizes and all that good stuff. We used batch sizes of eight, others can use higher batch sizes of 32 or whatnot. So that can drive different performance levels as well.
So, what you see is that our solution is again a fraction of the power and shows best-in-class performance per watt. Performance per watt is in our DNA at Qualcomm. We’re really leveraging that technology from the mobile side and bringing it over into edge and the data center. And it's resonating really well in the market and we’re really excited about the opportunities that we're seeing.
So, what does this all mean?
Back in March, AMD announced their next generation epic, what they call the Milan 7003 solution. As part of that announcement, a new gigabyte, G292 Z43 server solution was also announced. This server solution works very well with our PCIe card, our half-height, half-length card, capable of getting up to 400 TOPS in that form factor.
In this new two-use server, you can actually fit 16 of these Cloud AI 100 cards into that at 400 TOPS each. This means that cumulatively you can deliver 6.4 Peta OPS, that's 400 TOPS by 16 cards per server and 19 servers per rack. We think that this is amazing performance that’ll unlock new and exciting experiences that demonstrate how our low-power performance can scale at the server and the rack level.
To provide a little more context aligned with ResNet-50, this translates to more than 6 million images per second on one server rack for ResNet-50, and this kind of AI performance can surely enhance, extend, and scale AI experiences to the world. So for me, this provides a real concrete example of how our low-power performance scales at the server and rack level.
Key takeaways
Doing AI at the data center level might be a new area for Qualcomm, but we're really leveraging a lot of the heritage and history of the company with our mobile DNA, low-power processing DNA, and over two decades of AI research and commercial products that we're bringing to market.
And that makes us really excited about addressing this market in a different way, from a performance per watt story, and leveraging our mobile technology.
This is driving significant traction today, and we're looking forward to sharing more exciting launches to come in the second half of this year.
And we're very committed to this space. You’ve seen the MLPerf results, that's feedback I got in the middle of last year. They said, “Hey, John, this looks really compelling but we want to see your MLPerf results.”
And if you look at MLPerf, we're excited to be part of it. It’s a really great forum, but it's a lot of work. We took the risk and put our results out there. Not everyone in the industry is doing that, but we're really excited to do that and we continue to do it.
So as I said, there's performance and latency, and leadership really translates to what a customer’s expecting from us, and we're seeing the interest so we're really excited about that.