Korean web giant Naver recently unveiled a new family of large language models (LLMs) named HyperCLOVA X. These models are designed to excel in cross-lingual reasoning across Asian languages, potentially paving the way for the development of regional sovereign large language models.
Technical insights on HyperCLOVA X
In its announcement, made in Korean, Naver highlighted the model's superior performance in languages beyond Korean, including English.
A technical report available in the arXiv open-access journal emphasizes HyperCLOVA X's broad linguistic capabilities, suggesting it could serve as a model for countries interested in developing their large language models.
Training data and multilingual performance
The HyperCLOVA X models were trained on a mix of Korean, English, and other languages, with Korean content constituting about a third of the data.
This focus aims to enhance the models' performance in Korean while also considering the unique aspects of the language's grammar. Naver reports that this approach has yielded models proficient in both Korean and English and capable of understanding and translating languages it was not directly trained on.
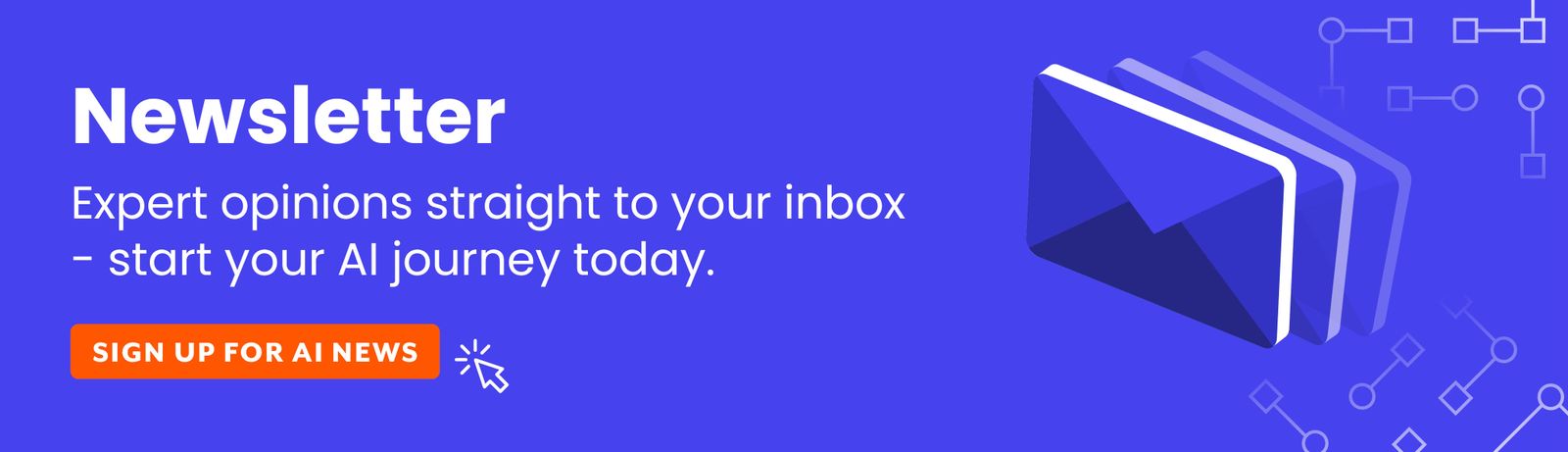
Sovereign AI and the need for localization
The report also discusses the concept of sovereign artificial intelligence, which is seen as crucial for national data security and reducing reliance on foreign technologies.
This push towards sovereign AI is partly a response to the overrepresentation of English and North American content in the training data of current LLMs, which limits their effectiveness with non-English languages and cultural contexts.
Future plans and enhancements
Looking ahead, Naver aims to develop specialized AI models for various regions and countries. The company is also exploring ways to enhance HyperCLOVA X's capabilities, including multimodal processing for handling text, images, and audio more seamlessly, and integrating external tools and APIs to access specialized datasets and services.
Want to know more about LLMs? Read the article below: