Another month and another news roundup - this time we’re taking a look at what happened in the world of artificial intelligence (AI) in the month of May. Last month, we took a peek at how AI is helping to protect Hawaii’s biodiversity, how machine learning can help to see into volcano eruptions, and more.
From monitoring the health of coral reefs to an automated blood collection device and much more, there hasn’t been a dull day in May!
In this article, we’ll take a look at a few AI, machine learning, and deep learning news, including:
- Artificial intelligence tool helps to determine ecosystem health
- Vitestro unveils autonomous blood drawing device
- Novel machine learning technique to develop new drugs
- Machine learning techniques for better characterization of long COVID
- A deep learning technique solves one of the tough challenges of robotics
- Neuromorphic chips “up to 16x more energy efficient” for deep learning
Artificial intelligence tool helps to determine ecosystem health
According to a study by the Great Barrier Reef Marine Park Authority, around 91% of Australia's Great Barrier Reef suffered coral bleaching in the summer of 2021-2022, due to heat stress from the rise in water temperatures.
Using sound, a new AI tool could help to determine ecosystem health. The research from UK researchers was published in Ecological Indicators, focusing on using AI to determine ecosystem health by studying the soundscape of Indonesian reefs. The study results indicate that the tool could use sound to learn the “song of the reef” and determine its health with 92% accuracy.
With the multiple threats that coral reefs are facing, it’s important to monitor their health and invest in conservation projects. Visual and acoustic surveys tend to rely on labor-intensive methods being limited due to the patterns of the reef lifeforms and the sound complexities.
Using machine learning algorithms, a computer can pick up on sounds and patterns that the human ear can’t, offering faster and more accurate results about the reef’s health.
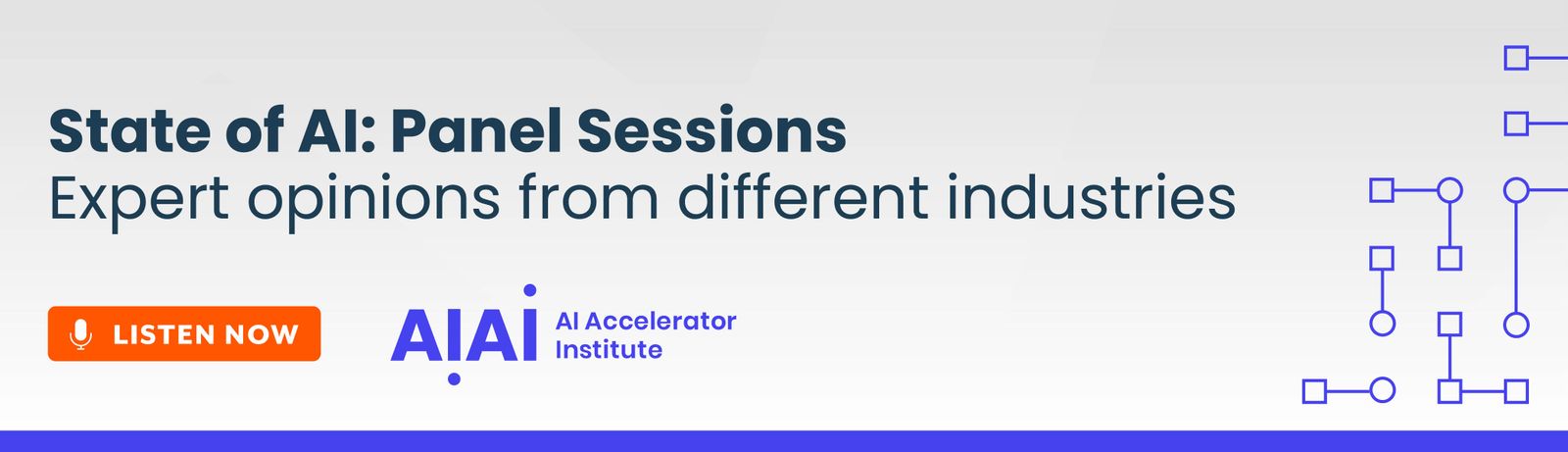
Vitestro unveils autonomous blood drawing device
The Dutch medical robotics company, Vitestro, showcased the world’s most advanced blood drawing device, which combines AI-based, ultrasound-guided 3D reconstruction with robotic needle insertion. It’s anticipated that EU-market instructions are made in 2024.
A healthcare shortage is a factor behind this development and phlebotomy (or blood collection), is the most common invasive medical procedure. If automated, it can help alleviate the healthcare system. Vitestro’s device allows for almost complete automation of the pre-analytical phase, meaning it could reduce high blood test error rates.
The company will first implement its device in outpatient phlebotomy departments, giving patients the option to choose between the device or a standard method. From Vitestro’s clinical trials, patients of all ages seem to have a high degree of willingness, openness, and enthusiasm in adopting this innovation.
Novel machine learning technique to develop new drugs
A new approach, which constrains a machine learning model to only suggest molecular structures that can be synthesized, has been brought forward by researchers from the Massachusetts Institute of Technology (MIT) in the US. This approach makes sure that molecules are composed of materials that can be bought, with the chemical reactions between materials following the laws of chemistry.
Other methods can take several minutes to evaluate molecule synthesisability. However, this new approach takes less than one second to propose a synthetic pathway. It simulates the process of synthesizing, being given a set of viable building blocks that can be purchased and a list of valid chemical reactions.
The machine learning model builds a tree by choosing building blocks and linking them through chemical reactions, one at a time, resulting in the final molecule. Researchers input a complete molecular structure, a set of building blocks, and chemical reactions to train the model, which then learns to create a tree that synthesizes the molecule. Hundreds of examples later, the model learns to come up with synthetic pathways on its own.
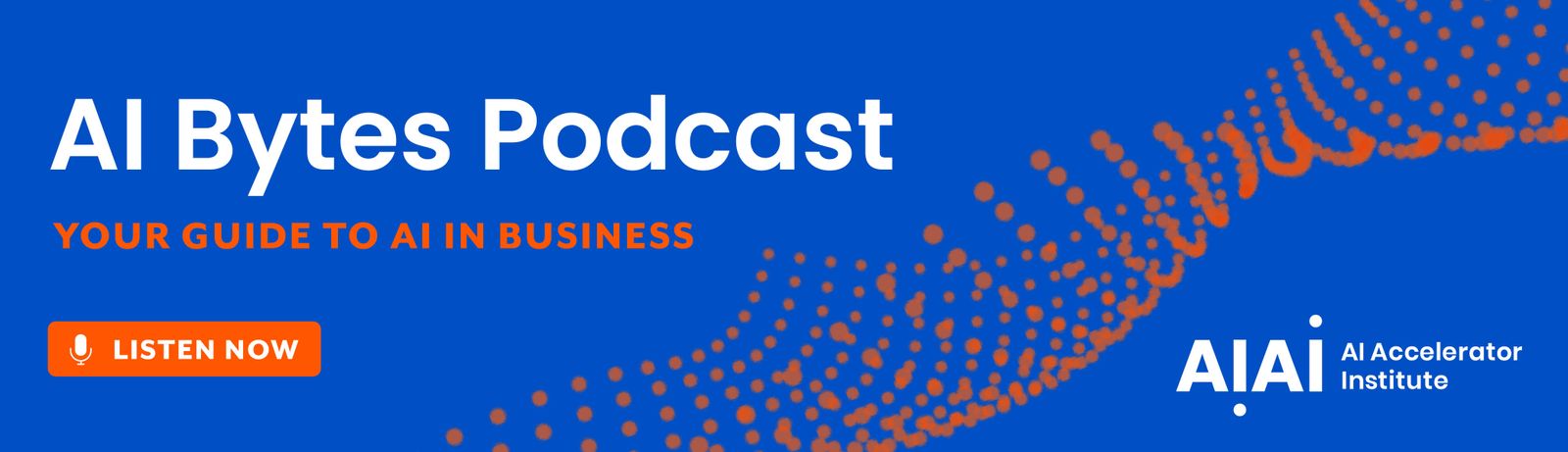
Machine learning techniques for better characterization of long COVID
By using machine learning techniques, scientists analyzed a large collection of electronic health records (EHRs) that are available for COVID-19 research, in order to better identify who suffers from long COVID. The team used data from the National COVID Cohort Collaborative (N3C) to find over 200,000 likely long COVID cases as of May 2022.
Characterized by a variety of symptoms, long COVID includes fatigue, headaches, shortness of breath, “brain fog”, fever, and other issues. These symptoms can last for months. They’re difficult to identify due to being similar to other diseases and conditions. Having a better view of long COVID could help to improve both diagnoses and therapeutic approaches.
Researchers looked at patient demographics, diagnoses, health care use, and medications in the health records of 97,995 adult COVID-19 patients from the N2C database. This information and data on 600 long CPBID patients from three long COBID clinics helped to create three machine learning models to identify long COVID patients. The models look for patterns in data to help researchers understand vital characteristics in patients and to better identify the condition in individuals.
A deep learning technique solves one of the tough challenges of robotics
AI systems and robots face difficulties when manipulating deformable objects. Developed by researchers at MIT, Carnegie Mellon University, a new deep learning technique shows promise in making robotics systems more stable when handling deformable objects. The technique, DiffSkill, uses deep neural networks to learn simple skills and a planning module to combine these skills to solve tasks requiring multiple tools and steps.
For an AI system to handle an object, it must detect and define its state and predict how the object will look in the future. A deep neural network can detect rigid objects from different angles with a good set of training examples. However, the same can’t be said when it comes to deformable objects.
Inspired by PlasticineLab, DiffSkill is a framework where the AI agent learns skill abstraction by using the differentiable physics model, composing them to achieve complicated manipulation tasks. It’s got a “neural skill abstractor” and a “planner”, generating training examples for the skill abstractor with a differentiable physics simulator.
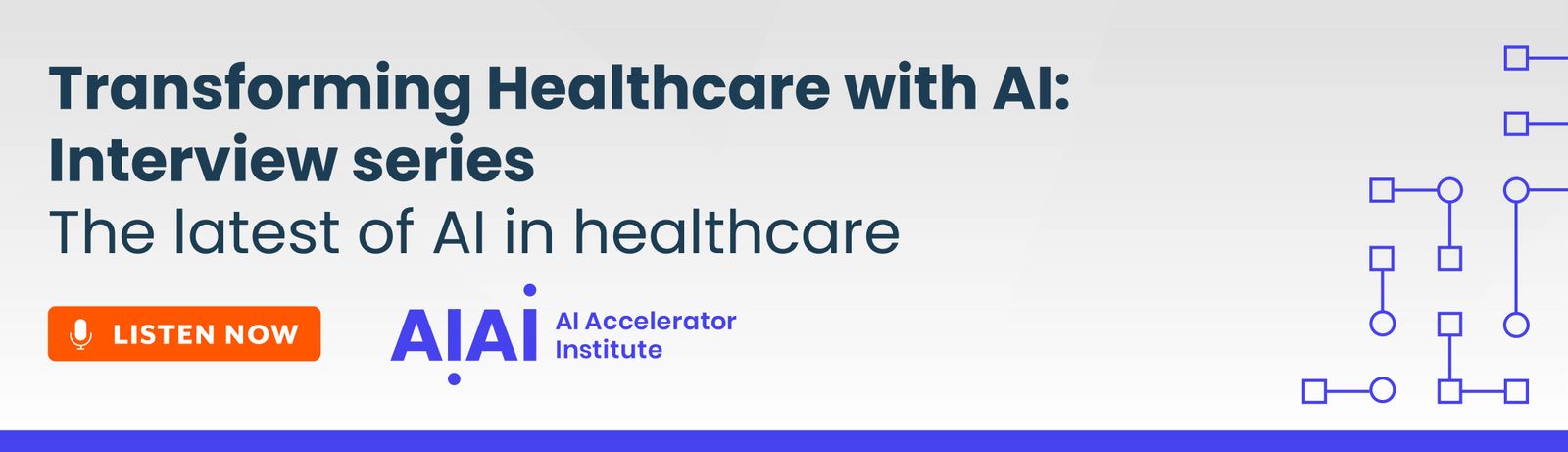
Neuromorphic chips “up to 16x more energy efficient” for deep learning
A study carried out by the Institute of Theoretical Computer Science at the Graz University of Technology (TU Graz) in Austria used Intel’s Loihi 2 silicon - a second-generation experimental neuromorphic chip with around a million artificial neurons.
Published in Nature Machine Intelligence, the research paper A Long Short-Term Memory for AI Applications in Spike-based Neuromorphic Hardware claimed that the Intel Loihi 2 silicon chips are up to 16x more energy efficient in deep learning tasks when compared to non-neuromorphic hardware.
TU Graz claims that it’s the first time that this energy efficiency has been demonstrated experimentally, which is important due to deep learning models being subject to worldwide AI research for real-world application deployment. Energy consumption of hardware needed to operate models is a big obstacle, which makes this announcement exciting.
Download our AI Chips of Choice 2022 report and have a look at the best computer chips from the best semiconductor companies on the market.
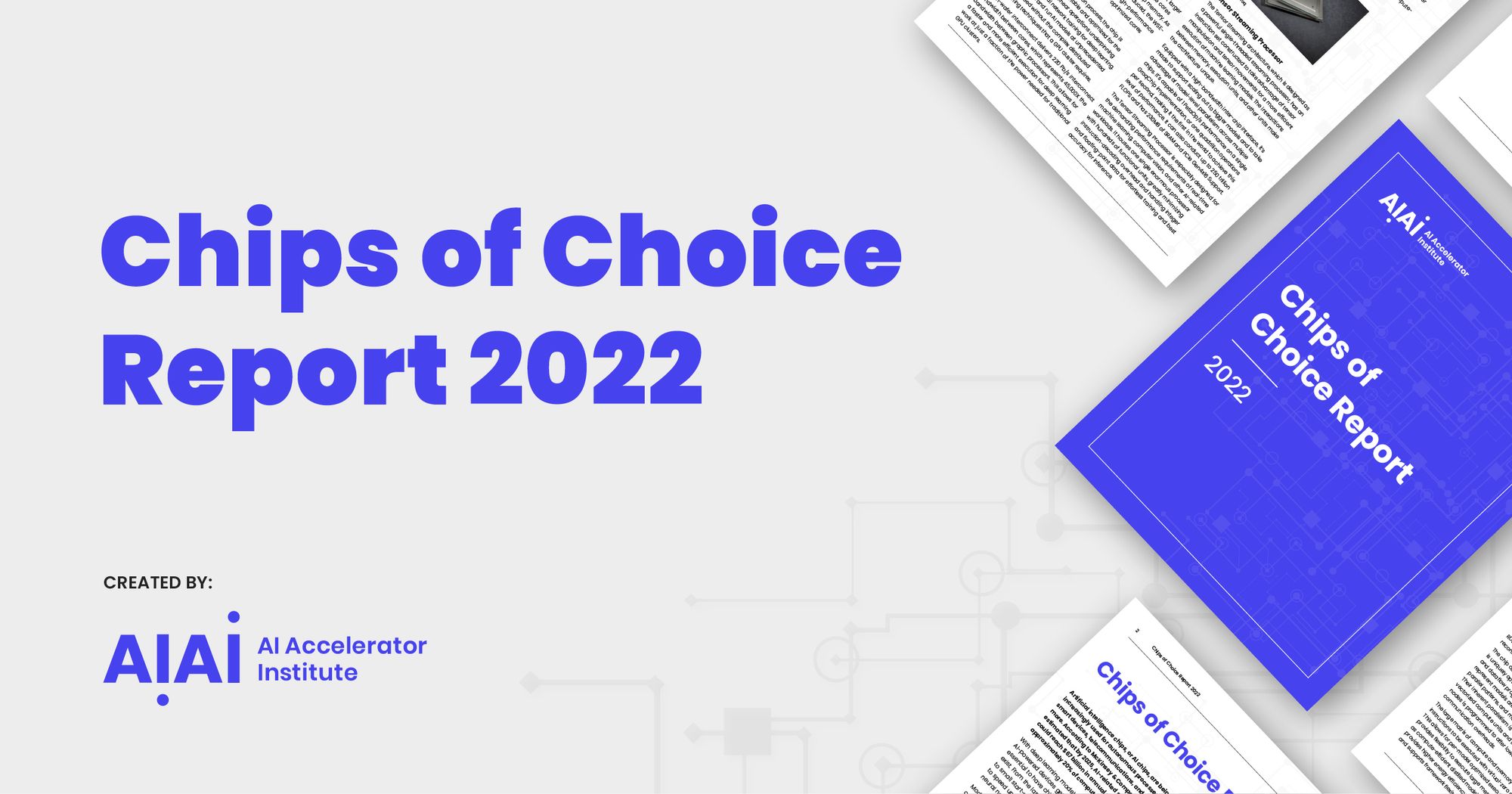