By 2050, there will be more than two billion additional people on the planet, requiring 50% more food from the same agricultural footprint upon which we are producing today.
At the same time, climate change is leading to more volatile weather and generally warmer conditions. And this comes at a cost. Experts are estimating that by 2050, we will have lost 17% of our annual harvest from our four key crop groups, core screens, oilseeds, wheat, and rice from climate change.
And when you look at the combination of population growth, and a fixed footprint of arable land, this translates to a 20% reduction in the area in arable land per person by 2050. This means that we will have only 1700 square meters of arable land per person, or about the size of a quarter of a soccer field per person for food production for their entire lifetime.
At the most basic level, this means that we need to be able to secure a sufficient supply of food while using our natural resources more efficiently and responsibly. And this is one of the biggest challenges that we as humanity face.
In this article, you’ll have an overview of:
- Bayer Crop Science’s breeding process
- How past plant breeding processes were manual with limited datasets
- Field automation and how data is essential
- How optimizing initial crossing increased the accuracy and diversity
- Precision breeding as Bayer Crop Science’s next big moonshot
As well as an in-depth examination of high throughput phenotyping, including, but not limited to:
- Automation
- Early season imaging metrics
- Plant height with LIDAR
- Late-season metrics
- Monitoring stalk health
- Image processing pipeline
Sound good to you? Let's get stuck in.
Bayer Crop Science’s breeding process
One way to overcome this challenge is to continue to improve seed varieties through plant breeding. Simply described, plant breeding is taking two existing varieties of seeds and crossing them to generate multiple progeny. These progeny are then tested in various environmental and agronomic conditions. And based on the performance that they show, we advanced the best performing varieties to subsequent years of testing. Each year, we continue to test them under diverse conditions, and then eventually commercialize the best performing varieties.
This process will take about eight to ten years on average. Over the last few years, Bayer Crop Science was able to improve the genetic merit of different varieties of seeds. Due to the genetic improvements and gains, they’re able to produce much more today using the same resources as in the 1940s.
Bayer Crop Science starts by crossing existing varieties, and in a commercial breeding pipeline, there are tens of millions of potential combinations that can be created. The different varieties are then evaluated in the fields and in the labs.
At the beginning of the pipeline, there are lots of decisions to make. As the pipeline advances, the number of decisions is reduced but the cost of each decision increases. This means that, in the earlier parts of the pipeline, a wrong decision made in terms of advancing a genetically weaker variety will cost only a fraction of the cost of taking that same decision, at a much later stage of the pipeline.
Past plant breeding progress was based on manual processes and limited datasets
The way these advancement decisions are made is by analyzing all the data that has been
collected on these various different entities. There are extensive field testing programs today, through which different kinds of data points are collected across multiple years.
In the past, collecting this data was a highly manual and labor-intensive process. The seed varieties were packaged in various envelopes, and these envelopes were then shipped to the various locations where they needed to be tested.
These seeds were then planted manually at these locations, and at different intermediate growth stages. Agronomists would manually walk the plots and collect all the needed data points and would store them in simple ways like Excel sheets. Statistical techniques and calculations were then performed on these data points, to decide what varieties to advance to the next stage of the testing and which not to.
However, due to the manual nature of this process, there was a limitation on the amount of data that could be collected. As a result, the decisions that were made were based on these limited data points and weren’t very accurate. The biggest drawback of this process, however, was that because of this limited data, the scope and the potential to predict the future performance of a variety was very limited and biased.
High throughput phenotyping and automation
In the past decade, there have been rapid technological advancements made in the areas of machine learning, artificial intelligence and deep learning which, coupled with the advances that have been made in sensor technologies, edge processing and embedded automation systems, have turned around the breeding game and resulted in a different approach.
Bayer Crop Science are now able to conduct high throughput phenotyping at scale, which has become the foundation of their breeding programme. Generally described, phenotyping is all the measurable traits and characteristics of a plant observed in the field. As field trials are conducted, there are several rates of interest to measure over the course of crop growth.
Things to keep in mind:
- Seed health
- Planting conditions
- Soil characteristics
- Topography
- In-season weather
- Pathogen presence
- Growth patterns and stress
- Yield
For example, for a corn plot, apart from physical characteristics, such as plant height, ear height, leaf canopy, etc., other characteristics need to be measured, like yield stock and route lodging to know whether a plant can stand in the field or not. Additionally, the environmental management characteristics under which a plant grew, like precipitation, soil conditions, and more are also measured, alongside measuring management practices such as tillage and irrigation in-season weather patterns is also important.
High throughput phenotyping: early season imaging metrics
After highlighting the type of data needed for measurement and phenotyping, the next step is how the data is collected through advances in phenotyping and automation. The first example deals with early-season growth milestones.
Uniformity measures the variance of canopy cover, which is the percentage of green vegetation in a given plot, within a plot, while stand is the count of detected plants within a plot. These metrics are computed utilising RGB images captured with UAVs (or unmanned aerial vehicles) flown during early vegetative growth stages at about 40 to 60 meters of height altitude.
Plot uniformity is then calculated by dividing plot images into smaller regions and calculating the coefficient of variation of canopy cover over them, being great for capturing the plot quality. Non-uniform plots due to emergence or plant size will have a higher canopy cover compared to uniform plots.
Stand, on the other hand, is calculated by detecting the peaks of one of the profiles of the crops.
High throughput phenotyping: plant height with LIDAR
Data is captured on a 3D point cloud when measuring plant height using LIDAR. This 3D point cloud data undergoes outlier removal, classification, and interpolation to generate surface and elevation models. Plant grids are mapped and aligned to extract plot extents from each surface, and metrics are computed for each plot. Before, standard manual plant height collection used one data point per plot, but LIDAR generates up to 200 data points per plot.
These measurements show a greater than or more than 90% correlation with manual measurements. And this is critical because accurate plant height measurements improve the estimation and yield accuracy by being able to compensate for neighboring effects.
High throughput phenotyping: late-season metrics
The key is to collect and analyze spatial-temporal data, which is data that has been collected over multiple time points, and monitor and use that data to monitor and model the growth pattern. NDVI, or Normalised Vegetative Differential Index, which measures the extent of greenness in a crop, is a common metric used to measure and model plants in essence or plant decay.
The growth and senescence patterns of known germplasm, whose behaviours have been studied by breeders and economics for years, are modeled, becoming the basis for predicting the growth patterns of the newer germplasm. A combination of RGB and multispectral sensors is used to capture this data.
High throughput phenotyping: monitoring stalk health
To monitor stalk health, reporting the percentage of healthy stalks per plot is essential. This is done by imaging the plot from a combine or a tractor, and then using those images to generate metrics on the health of the stalks by using a machine learning model.
These images are separated into groups by plots by using applications that incorporate GPS and motion sensors to accurately determine where in the field these images came from. These images are then run through a model, which has been manually trained with manually labelled images that have been provided by plant health teams in order to provide the stalk health percentage.
High throughput phenotyping: image processing pipeline
Generating high throughput phenotyping data requires a robust image processing application and robust image processing pipelines at scale, which can typically handle large volumes of data, some of which are processed at the edge.
These pipelines are able to perform a combination of tasks such as spatial validation, tile rectification, which involves both blur and exposure correction and image registration, followed by geo-referencing, image teaching, calibration and QC of the ortho mosaic. Metrics are then extracted to the nature and the purpose of the phenotyping effort.
Field automation: data is essential to run highly automated, centralized breeding operations
Extensive combine and planter automation software has been developed using vision techniques to control processes within the equipment and to also be able to provide common user interface environments for their respective platforms to enable GPS logging of events and to standardize data handling.
For example, within Bayer Crop Science’s seed processing applications, they’ve used sorting machines that can be set to classify and sort seeds based on several metrics. Through the experimental design of their fields, Bayer Crop Science has also used these vision systems to measure how well hardware equipment is keeping grain separated between the plots.
This provides an insight into how well equipment is performing, as well as how accurate other plot-based measurements are with respect to a plot-to-plot carryover, thereby allowing Bayer Crop Science only to use a minimum amount of plots to test for statistical significance.
Optimizing initial crossing increased the accuracy and diversity
Bringing all this data together drives decisions differently. Collecting data used to be a very manual and labor-intensive process even just a few years ago. As a result, the size and the accuracy of the data used to advance genetics to the next stage of the breeding pipeline were very limited and sometimes resulted in poor decision-making.
The process itself was also extremely manual, which added more complexity. Through automation and high throughput phenotyping, Bayer Crop Science has significantly increased the collected data in fields, labs, and other facilities like greenhouses, while observing higher accuracy of data points.
As a result, great datasets can be built to train advanced machine learning models that are assisting in the new product development and advancement decisions. This translates to better products brought to pharma clients and customers.
Precision breeding as Bayer Crop Science’s next big moonshot
One of the key elements of the breeding process is to create a genetic variation through crossing and selecting the best performing entities among them, and then advancing those to the next generation.
A key shift that needs to be made for the future is to transition from selecting the best to designing the best. This means that, rather than depending on the recombination events that occur during crossing, Bayer Crop Science wants to be very intentional in creating a genomic variation that will result in the desired outcome.
Making the shift from selecting the best to designing the best will be extremely challenging. It will require an understanding of which regions in the genome are responsible for the phenotypes that are observed in the field under different environmental conditions. In order to understand these interactions, more data sets need to be built to increase scale resolution and accuracy.
These datasets can then be used to build models that help optimize this transition to designing.
Bayer Crop Science has billions of diverse data sets across genomics, imagery, environment, weather data, machine data, and more, using these data sets to solve some of the most interesting, challenging and impactful problems within agriculture.
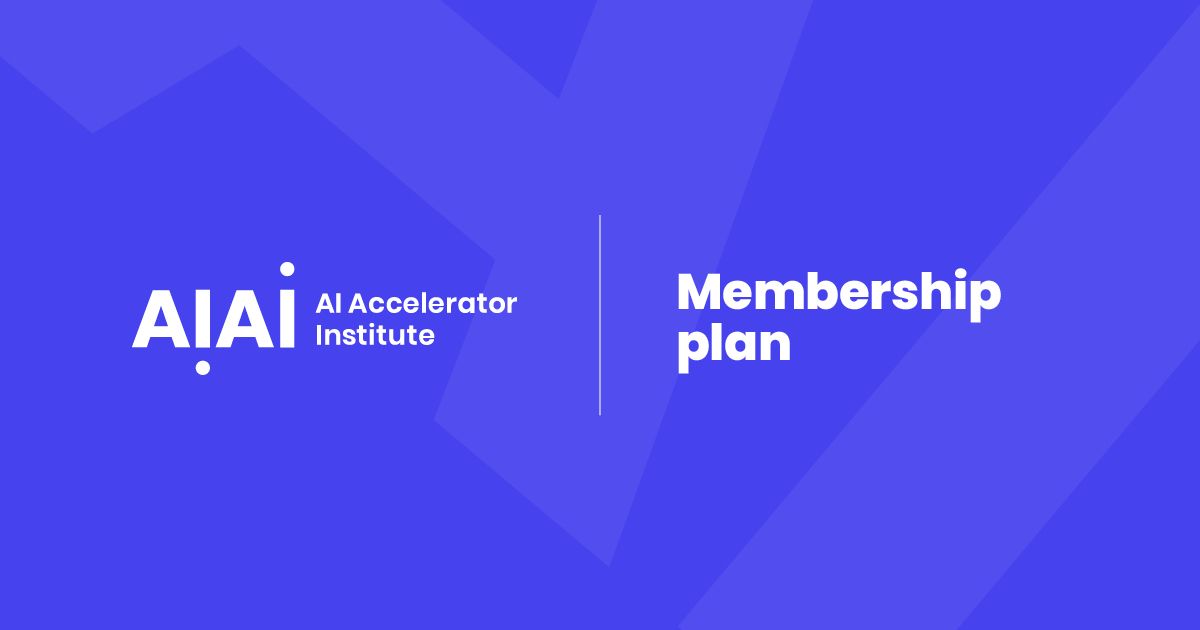
Interested in more exciting talks on how image processing and computer vision are shaping the future of industries like agriculture? Then why not become a member today!