Industrial AI is becoming an essential tool to drive business value. Knowing how to combine software and hardware AI capabilities at the edge to deliver this value is an important step for the streamlining of machine components.
The application of AI in industrial use cases completely revolutionizes manufacturing by disrupting the supply chain and improving automation processes. In this article we’ll cover:
- Drivers and dynamics in the industry
- AI integration in business processes
- What the ‘digital twin’ is
- Aviation customer outcomes
- Continuous learning and improvement
- A look into additive manufacturing
Industry value: drivers and dynamics
The drivers:
- Increased productivity: How to get better asset productivity?
- Faster growth: How to find ways of generating more revenue?
- Risk-managed adaptability: How to generate revenue in a way that manages business risks as the world changes?
- Improved safety: How to do it safely?
The trick is to not just focus on one or two drivers but to do them all at once. By doing so, there are certain dynamics that happen:
1. Deeper customer engagement
In addition to dealing with customers, there’s now a need to deal with customers’ customers. This involves dealing with the airline companies, the people who fly on the jets - because without them there’s no revenue stream - and the electricity companies’ customers, who like to know they’re getting renewable energy and how they manage it themselves.
2. Blurring markets and government influences
There’s also the actual landscape, which is a competitive one. There are new competitors emerging, like Amazon. In addition to his focus on retail, Jeff Bezos created a company in 2000 called Blue Origin that builds rockets.
He invested a billion dollars until 2011, and from that year onwards, he made a billion dollars a year. His rockets were chosen for the Vulcan program, a combination of Lockheed and Boeing.
Elon Musk, for example, has gone from PayPal to aerospace with SpaceX, Tesla, and more. In addition to this new competition, there are also government tariffs impacting the landscape.
3. Digital: higher capabilities at a lower cost
The world has evolved from digital - with humans engaging with information, services, and other humans - to now, with humans engaging with machines and machines engaging with machines. General Electric (GE) has a robot inspecting wind turbines, for example, which is a great example of machines engaging with machines.
All of these dynamics are driving the financial drivers to an installed base that's on this planet for years to come. These engines last anywhere from 20 to 40 years, so how do we use AI to actually allow us to meet these financial drivers?
Creating value: AI integration in business processes
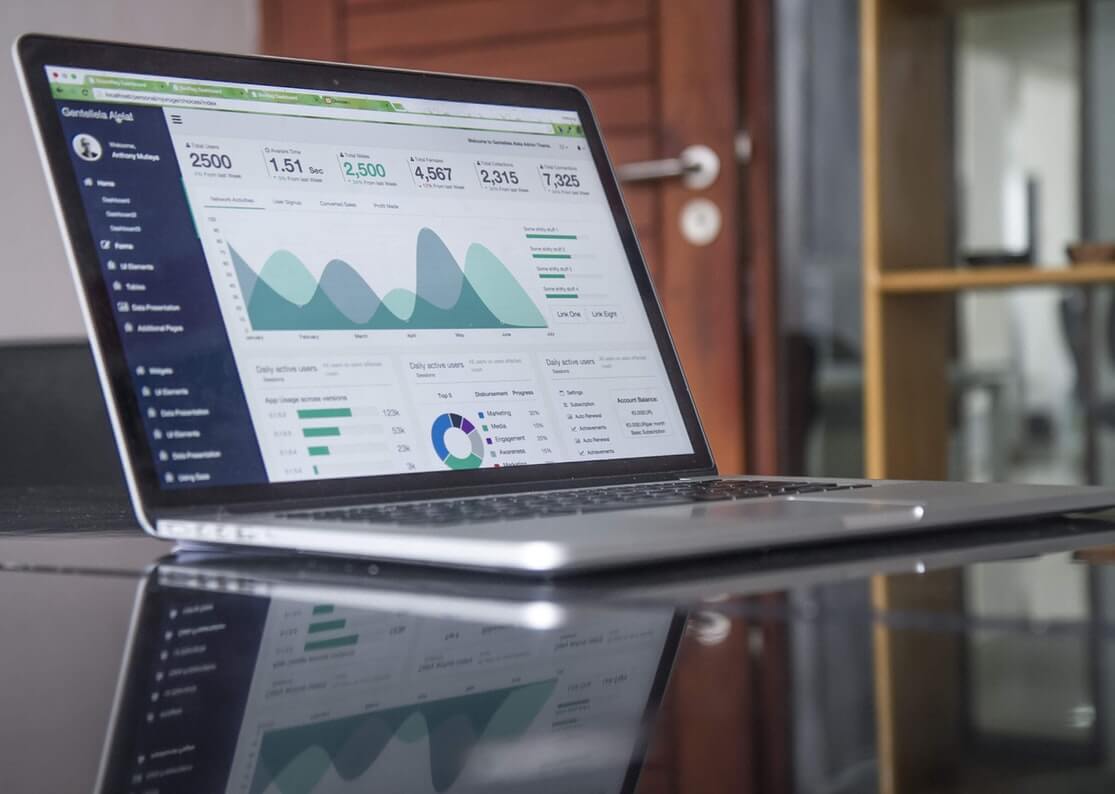
GE spent some time looking at the consumer space, that space that has been using AI for a number of years, and decided to figure out the best practices. They decided to take a look at them and evolve them, innovating around them.
Around six to seven years ago, they found three companies (Amazon, Apple, and Google) doing unusual work that made them leaders, while all using the same method of digital transformation. Looking at their revenue and market share, which are important factors when it comes to leadership in a business sense, you see how they’ve impacted the market.
In 2018, Apple made around $265 billion, Amazon made $236 billion, and Google made $135 billion. These companies range from 24 to 46 years old, and it took GE 102 years to get to $100 billion.
The Amazon example
Amazon, for example, (but keeping in mind that Google and Apple also use the same techniques) started off by creating a model of one.
Amazon started off by trying to get demographic data: if this group likes this book, then maybe someone else would like this book as well. Amazon quickly went from demographic data, or to data on an individual rather than groups and individuals.
They looked at what an individual bought, when they bought, what ads they looked at, at what reviews, what they did on Mother's Day, and where you sent the information. Amazon collected all of that data from 300 million of their users and created a psychographic model, which is a model on how you buy and when you buy.
With that model of one, they apply the AI. So they're looking at things like segmentation. If you belong in a segment and a segment has a book, then they offer you that book at a discount. Amazon looked at prediction - if they see you looking at these ads and these product reviews, then they offer you these skis, for example, at a discount. If you buy the skis, then it’s profiling, because they can predict, after the AI profiles you, that after the skis you’ll want a helmet. So you’re offered a helmet at a discount.
In summary, Amazon looks at segmentation, profiling, and prediction with AI techniques. By doing so, they created a P&L of one. They look at the model and they focus that model on you with the right ads, and they create the ability to generate a certain type of P&L.
From a model of one to a P&L of one, then Amazon creates a platform for all, so they can reuse it a lot quicker and cheaper. Going from books to general retail to web services, they then amped the model to offer the Kindle, in order to get information from customers very quickly. Then they placed Alexa in homes, which people speak to and allow to collect information, making it a much better model of one, all going through a P&L of one or to a platform of one.
Applying the same techniques at GE
GE took the same approach to see if they could apply the same techniques to their assets. With jet engines, for example, they normally collected aggregate and fleet information about it. Instead, GE started to collect information about an individual engine, individual asset, the design of the asset, the manufacturing of the asset, how it was serviced and operated, the environment the asset flies in, and more, to create a model of one.
Normally, GE applies fleet analytics - and how fleet analytics work, is based on assumptions. You assume a jet engine will fly between certain types of cities at a certain temperature and pressure in this contaminant level and, based upon how it’s operated, it’ll accelerate or decelerate at a certain speed for a certain distance based on those averages. You can then assume a certain part will last for 1,000 cycles or flights.
But instead of assuming, now GE can obtain the actual data and now the exact cities it flew between, the exact temperatures, pressure, dust contamination, and more, to know that a certain part can last 4,000 or 6,000 flights. This eliminates the need to bring it back every other thousandth flight. Instead, it can be brought in exactly when it needs to.
This means parts have a prolonged life cycle and the economics changed, and GE now has developed a P&L of one. Going from a model of one to a P&L of one, it can then be added onto a platform and apply the same thinking from jet engines to gas and wind turbines.
GE calls this the ‘digital twin’. It’s the capability of taking the deep knowledge of the physical asset, like the design, the manufacturing, the service, and the operation, and combining it with the data and AI techniques to create a living, learning model.
Extracting value: ‘digital twin’, a personalized, living, learning model
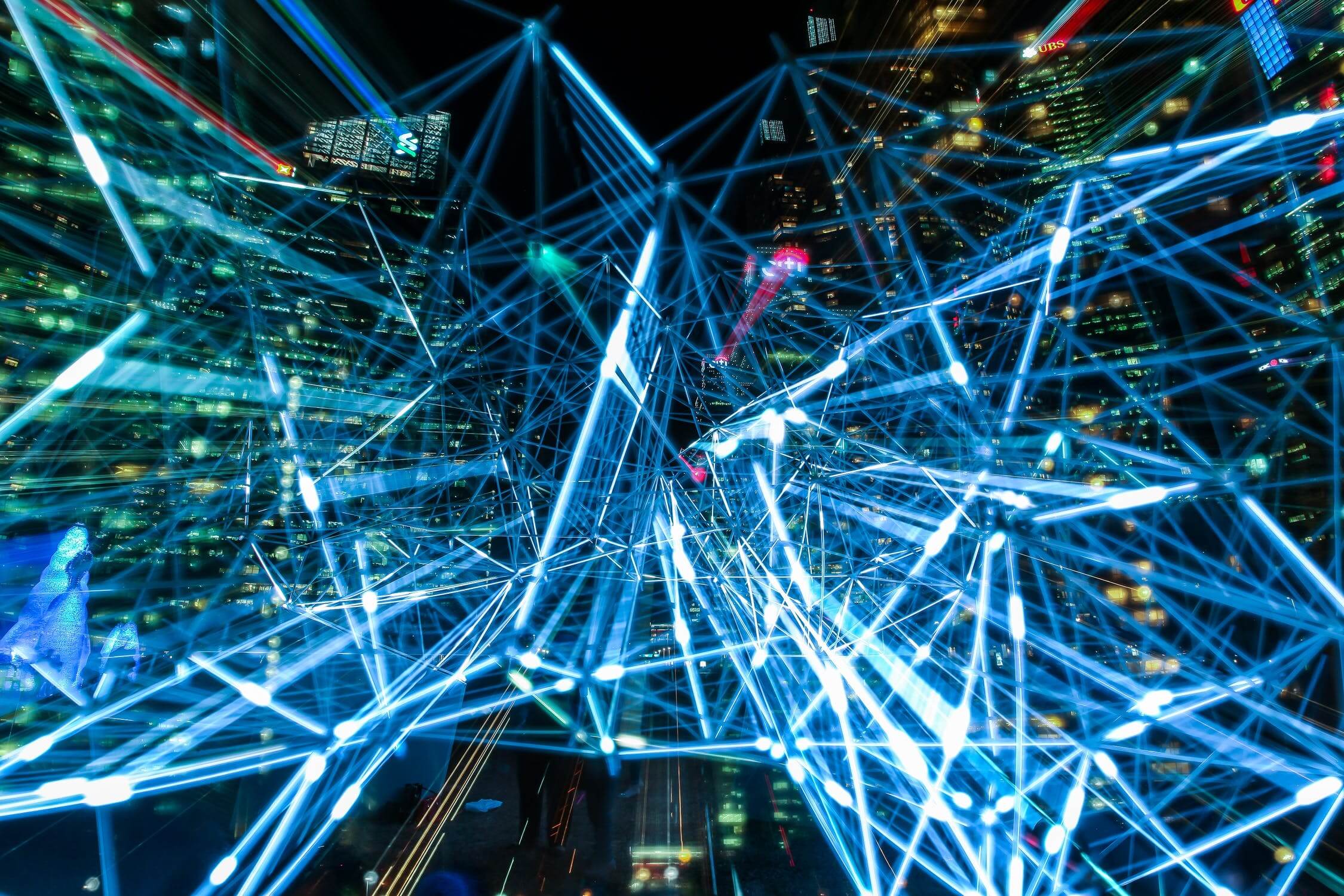
Models are usually created in the design phase for services when there’s a big problem. But these models can be continuously updated every time an engine lands to know how it was operated and where it was flown. This allows for fine tuning of the model, which becomes living.
Looking at an asset, and comparing it to others that fly the same way in the same pattern, allows GE to learn from what’s happening to them. So, this living, learning model combines the physical and the digital to provide insights on aspects like a part’s useful life cycle or efficiency.
Insights alone, however, don’t generate value. Action needs to be taken, meaning you have to make a decision on whether the part needs to be brought in for servicing or not. If you need to wash a part for better efficiency, that’s an action with value - as it shows in the fuel efficiency of the next flight - and this value can then be fed back into the ‘digital twins’, which get smarter every time.
Delivering value: aviation customer outcomes
Value can be extracted in three different ways:
1. Sufficient early warning
Planes usually have a compressor problem, which can happen at the gate, in which a light blinks on the dashboard. The flight is then either delayed or canceled, and all the passengers need to be removed from the plane. But with AI technology, GE can know 30 days in advance that an action needs to be taken, allowing for the plane to fly for longer and generate revenue.
2. Continuous prediction
Damage can also be predicted on a part, meaning GE knows exactly when an asset needs to be brought in, and not every other thousandth flight. They can say with certainty that an asset needs to come in every 4,000 flights, letting it have a greater availability so customers can make more money. The mechanic shop is also used more efficiently.
3. Dynamic optimization
With AI technology, GE can also know which assets should be flying certain routes. If you keep an asset with one set of engines flying on a route into a hot and harsh environment, that will wear out quicker. So, knowing how to balance assets in the right way lets you know if you need to lease fewer engines when they’re in the shop, which can help you reduce your leasing cost.
Over 2016 and 2017, GE built 1.2 million ‘digital twins’, generating $580 million of value. The power of the ‘digital twin’ is the ability for it to live and to learn. But how do you make sure it continually gets enhanced? GE finds ways to collect ground truth.
Enhancing value: continuous learning and improvement
The ‘digital twin’ is built to predict the life of an asset, like a coating. Thinking about the damage done on a blade, how can you predict what that damage will be to bring in the plane at the right time?
When the plane is brought in, an AI system looks at the blade and determines the damage on it. When a plane flies in hot and harsh environments, contaminants fall on the blade and eat the protective layer on the outside. If you can predict when the layer comes out, and what size the damage will be, then you’ll know when to bring in the plane.
When the plane is brought in, you need to know if the prediction was correct - that’s the ground truth. Usually, human employees clean the blade and put on a certain coating, before turning the light off and using a UV light to look for damage. The employees have to do this eight hours a day, five days a week for four years until they get good. And, statistically, Mondays and Fridays have the worst results.
Computer vision completely changes how this is done because there can be a system that figures out exactly where the damage is, gets the actual measurements of where the damage is, reflects on the fact that the last time the plane came in there was a specific amount of damage, and feeds it back into the ‘digital twin’. This lets the ‘digital twin’ get better, and a new coating can be designed to work better than the last one.
You can also create an ‘immortal machine’, which is the new journey GE is on. This is done by saying that the ‘digital twin’ isn’t providing any new information about the coating. And if you suppose that the right thing to do is to design a new blade, one that can withstand its environment perfectly, then you can create a blade that is longer living and has better performance.
A ‘digital twin’ is continuously getting smarter by learning from the environment and from people. Inside machines, just like with human bodies, we continually replace parts so they perform better, and this is how we create an immortal machine. But to do that, you're going to need additive manufacturing.
Additive manufacturing: disrupting engineering, manufacturing, and supply chains
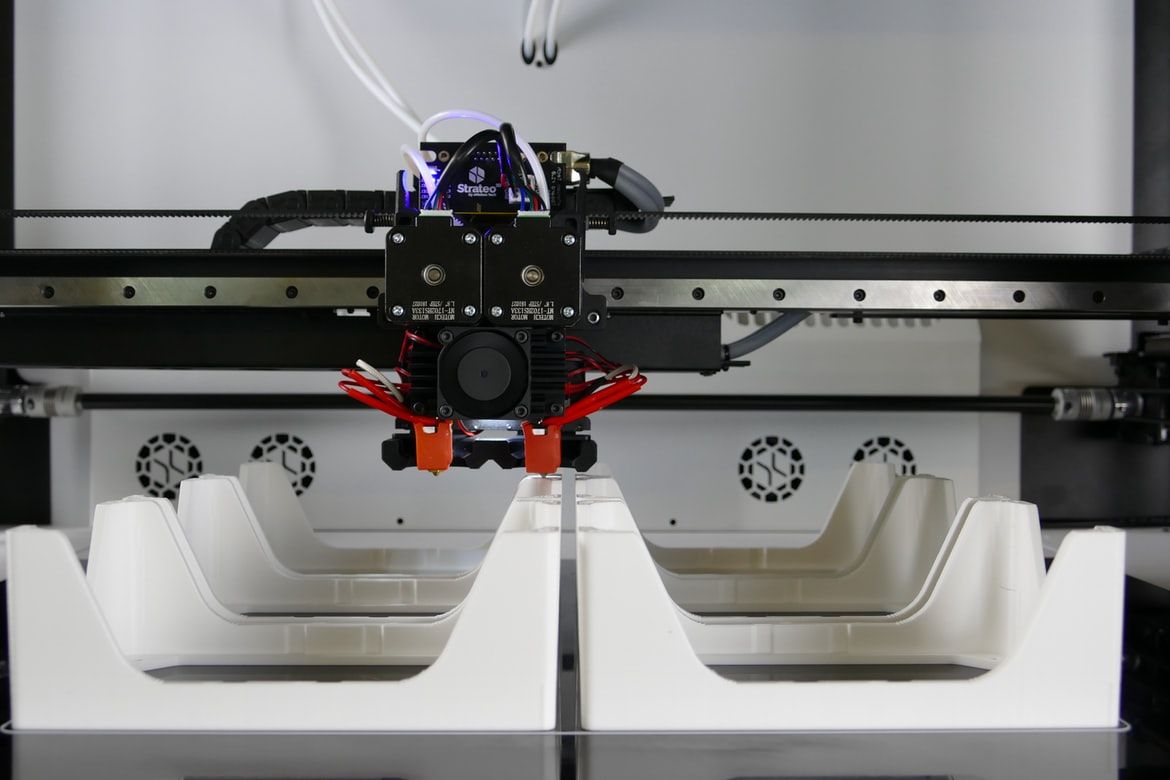
To enhance value at the right costs for each individual machine, you’ll need additive manufacturing and you’ll need to embed an AI capability in the machines. GE’s current status on where they’d like to go on the edge involves additive manufacturing.
GE both sells metal additive manufacturing machines and uses additive manufacturing for their own products. In aviation, for example, GE has a part from a 901 engine, which is a helicopter engine. It’s a successor to the T700, which has run GE’s Blackhawks since 1979.
The US Army wanted to achieve 25% more fuel burns, or better fuel efficiency, and get a certain percentage of weight out of the aircraft. GE was able to use additive technologies as a big differentiator in the competition to help them meet those requirements, winning the award.
In the past, the part that won the award would have been divided into 300 components. GE consolidated those 300 components into one, which was a big change for the aviation industry. But it’s not just part consolidation and achieving better performance. GE was able to reduce:
- The required 50 manufacturing systems to one.
- The 60 engineers needed six or eight.
Additive manufacturing isn’t just disrupting and making better parts. It's disrupting the supply chain and disrupting manufacturing at large. And it's disrupting engineering too.
The machines used by GE, laser bed powder machines, have a powder layer of metal. Then with a laser beam hitting the powder, metal is melted and it solidifies as the part is slowly built up, particle by particle. The area where the metal melts is where AI comes in to help.
Real-time analytics for industrial systems
What should be run from the cloud and what should be run at the edge?
The Nyquist criteria deal with how often something needs to be sampled so its behaviors can be seen, and things with slow or fast natural frequency have different reactions.
A wind turbine, for example, can be 130 metres long (bigger than a football field), and the natural frequency of a blade is about one hertz. Nyquist criterion says for me to see the movement of that blade, the dynamics of that blade, it needs to be sampled two times per frequency - or twice per second - which lets GE know it’s moving correctly.
To see how the blade is moving, the rule of thumb is to sample between 10 and 20 times per second. That means it needs to be sampled at 20 times faster than one second, which is 50 milliseconds.
So even for a really, really big system, it needs to be sampled 50 milliseconds so it can be observed, and so its behavior can be understood from a safety-critical point of view. From a UI point of view, there are other systems that are really fast, like electrical converters. In these, currents are moved around a circuit, and they’re in the 10-kilohertz range.
For additive manufacturing, the main frequency of that melt pool is about one kilohertz. For GE to be able to see what’s happening at the melt pool, they need to sample at about 10 to 20 kilohertz, or 10,000 to 20,000 times per second, which is very fast.
The next step is to look at the melt pool and understand a lot about it. In the past, GE could have done a thermocouple, speed, or pressure, each one being about 32 bits, which could mean hundreds of bits per sample. Looking at the very dense image of the system, a high-density camera translates to millions of pixels times 8 to 16 bits per pixel or tens of millions of bits per second.
After sampling fast enough to see it, the algorithms need to be run. Before, it was rule-based or based on simple models, but now GE is moving to deep neural networks to help them see all of this information. These neural networks need to be run at tens of gigaflops per sample.
This means that to see what’s going on in the melt pool, it needs to:
- Be incredibly fast.
- Have more data than ever before.
- Compute more data than ever before.
Real-time analytics on the edge
When a laser hits a powder bed, it’s too fast to see what happens. By slowing it down a thousand times slower than real-time, you can see how the AI system helps to understand the quality of the parts.
GE set up the system around their machine, using a camera that takes images on the order of 10 to 20 kilohertz, and ran lots of different parts with lots of different control settings to get training data for it, running around 10 million images through the system.
By using TensorFlow as their framework, they started off with an assumption for the neural net, which has been modified, and trained it on NVIDIA DGX (where they did the training). It took GE around 48 hours to run all simulations, and they’re getting 85% to 95% recall accuracy of quality parameters, like what’s happening in the melt pool, how wide it is, how much energy GE thinks it’s getting pulled into, and more.
Inference on the edge is the next step to streamline; when pulling in that much data and running it through that many calculations, it needs to be done much faster, from development to deployment on inference.
The first thing to figure out is how to get interoperability among the neural net frameworks and how to use tools and capabilities across them. The next thing needed is to be able to use these accelerators to be able to deploy it onto the hardware, so that processes are completed faster and there’s no need to learn a different deployment tool every time.
The last thing is to get to the wanted performance. GE is using Intel’s OpenVINO™ as a tool for deployment, running at about two kilohertz, or 2,000 frames per second in that entire process. However, they need to get to 10,000 to 20,000 to be able to see all the details.
If you can see the quality, then you should be able to control it to get to where it’s needed, which is a key step on the journey to get the ‘immortal machine’. A ‘just press print’ capability is the end goal, in which all you need to do is to press a button and a part comes out exactly like it needs to look.
Based on a talk by Colin Parris and Brent J. Brunell, GE Digital and GE Global Research.
Want more knowledge from AI experts? Why not sign up for our membership today?
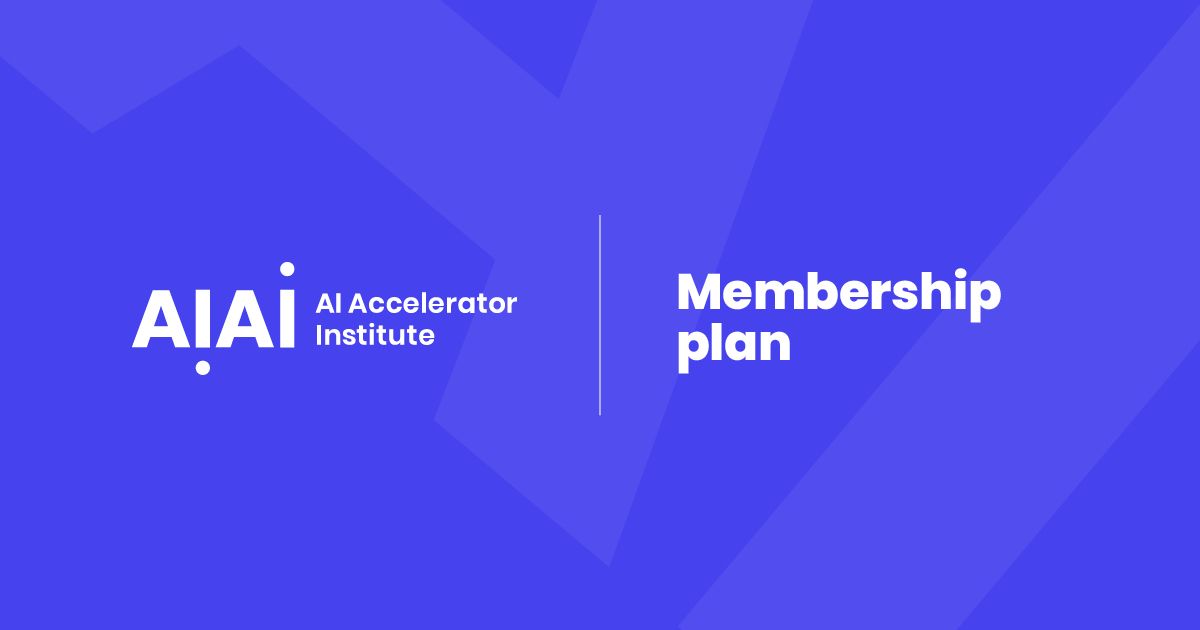