Coaches have used data science in sports to help improve their teams’ performance for decades. Big data helps to make real-time, split-second decisions, while sports analytics allows coaches to make big decisions with more confidence.
Computer vision is playing a big part in every sport, improving the fan experience, enhancing training sessions, and making sure referees make the right decisions.
In this article, we’ll go over a few use cases of computer vision in sports:
- Automated refereeing
- Real-time player tracking
- Match predictions
- Recruitment and scouting
- Automated journalism
- Ticketing
Want to know more about AI in sports? Read our article below:
Automated refereeing
The Hawk-Eye computer system is used in tennis and cricket to track the ball’s trajectory so that referees can tell whether it’s a fair or a foul ball. The system uses a camera network for tracking, comparing the trajectory of the ball to a virtual model of the playing surface.
As the system is accurate to a few millimeters, it’s a much more reliable resource than the referee’s human eye.
The NFL is also using a computer vision system, called Next Gen Stats, to track every player’s position on the field. This lets referees know whether they should call for a penalty or not.
Real-time player tracking
Player tracking involves detecting player positions at any time, and it’s a crucial element for coaches to help their teams improve their performance. Through tracking, they can instantly analyze how each individual player moves on the field and the team’s overall formation.
Computer vision applications can use segmentation techniques to help identify regions that correspond to players. The resulting data can then be augmented through machine learning and data mining.
When applications identify key elements in video frames or images, they generate semantic information to create context for the actions players are performing. They label semantic events like “one-two passes” in football so they can be used for advanced statistical analysis of both team and player performance. These insights are important to let coaches move players to their ideal position on the field.
Match predictions
Sports bettors have always tried to process data to better understand and predict the outcome of future matches so they can win a lot of money. Artificial Intelligence (AI)-driven algorithms can do it a lot faster, and more accurately than humans can. Algorithms might not be able to predict every single match’s outcome, but they can definitely get much closer than a human would.
Computer vision can determine ball possession time, for example. As its accuracy improves, algorithms will be able to successfully predict match results. But that’s not all; computer vision will also be able to collect and analyze data based on:
- Key passes leading to goal-scoring opportunities
- Number of passes between teammates
- Number of scored goals
…and more.
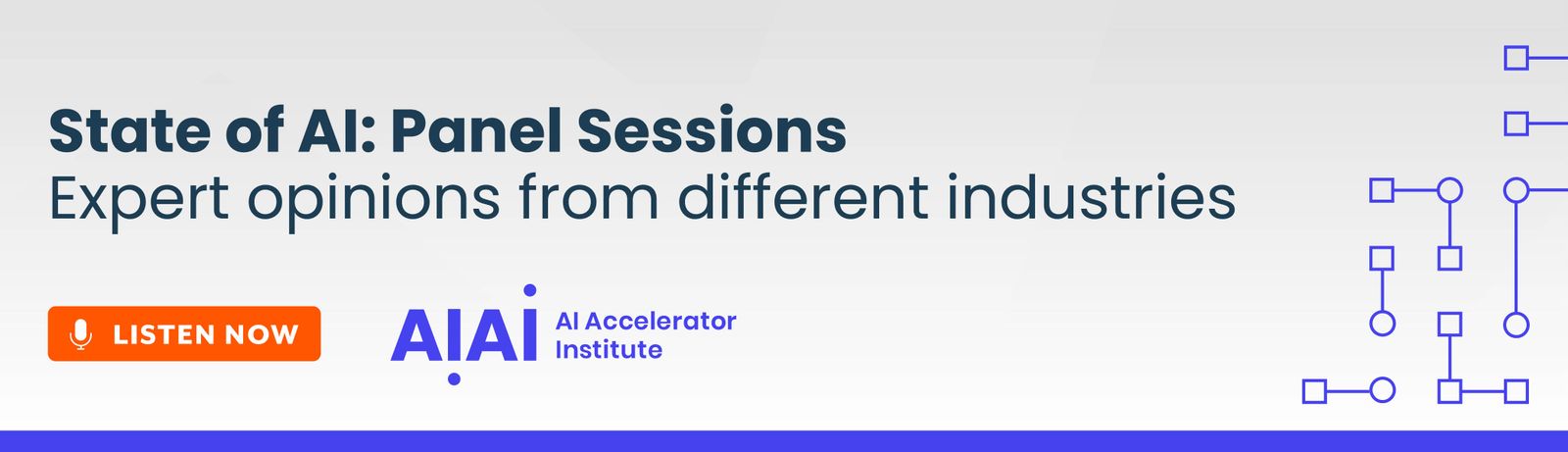
Recruitment and scouting
Today, computer vision tracks everything on the field of play, from runs in football to swings in baseball and more. This means teams gather terabytes of data on their players' movements and their bodies’ orientations while they play.
Machine learning algorithms use this aggregated data to evaluate player skills and their overall potential, allowing them to rank players in several categories. Teams can spot specific attributes to know future performance and make better recruitment decisions.
These insights help sports teams build more successful teams, buying untapped players for less money and then developing and selling them for a profit. Brentford had success with this strategy, acquiring striker Ollie Watkins for around US$2 million and selling him for US$35 million later on.
Automated journalism
Between statistics and data from football, baseball, tennis, and other different sports happening practically daily across the globe, there’s a lot for sports journalists to cover. AI can help ease this workload and make sure companies can cover a lot of events at the same time with little effort.
The AI-driven platform Wordsmith uses natural language to translate data from Minor League Baseball (MiLB) into narratives. It has helped the Associated Press (AP) increase reporting capacity to 13 leagues and 14 Major League affiliated teams.
AI bots can also write extremely accurate match reports covering statistics, key events, and other data. NDC, a Dutch regional media group, uses AI to cover 60,000 soccer matches a year – which translates to almost every single local match.
Ticketing
Elite sports teams still struggle to make sure fans can get inside stadiums on time. This is nothing new – it has been happening for decades, and there seemed to be nothing that could help solve the issue.
The Columbus Crew, however, is using facial recognition to help get fans indoors quickly. In partnership with computer vision software Wicket, fans can upload an image of their faces to the ticketing platform alongside the ticket barcode number.
Wicket devices then scan fans’ faces so they can pass without having to show their paper tickets. The team is also using Wicket to monitor crowd density inside the stadium, with the software sending staff alerts when specific areas get overcrowded.